Language of instruction : English |
Exam contract: not possible |
Sequentiality
|
|
No sequentiality
|
| Degree programme | | Study hours | Credits | P2 SBU | P2 SP | 2nd Chance Exam1 | Tolerance2 | Final grade3 | |
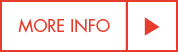 | 1st year Master Biostatistics - distance learning | Compulsory | 108 | 4,0 | 108 | 4,0 | Yes | Yes | Numerical |  |
|
| Learning outcomes |
- EC
| The student can handle scientific quantitative research questions, independently, effectively, creatively, and correctly using state-of-the-art design and analysis methodology and software. | | - DC
| ... correctly using state-of-the-art analysis methodology. | | - DC
| ... correctly using state-of-the-art software. | - EC
| The student is capable of acquiring new knowledge. | - EC
| The student is able to efficiently acquire, store and process data. | - EC
| The student can critically appraise methodology and challenge proposals for and reported results of data analysis. | - EC
| The student is an effective written and oral communicator, both within their own field as well as across disciplines. | - EC
| The student can put research and consulting aspects of one or more statistical fields into practice. | | - DC
| The student can put the consulting aspects of one or more statistical fields into practice.
| - EC
| The student is able to correctly use the theory, either methodologically or in an application context or both, thus contributing to scientific research within the field of statistical science, data science, or within the field of application. | | - DC
| The student is able to correctly use the theory in an application context, thus contributing to scientific research within the field of application. | | - DC
| The student is able to correctly use the theory in an application context, thus contributing to scientific research within the field of statistical and data science.
|
|
| EC = learning outcomes DC = partial outcomes BC = evaluation criteria |
|
The student should be familiar with basic concepts of statistics, such as: type I and type II error, hypothesis testing, and basic linear regression methodology. R software will be used for this course.
|
|
|
We will discuss rank test statistics for comparing two independent groups, two dependent groups, and the comparison of more than two independent and dependent groups. In the nonparametric regression part, we will start with Kernel Density functions, and then we introduce two basic approaches on Nonparametric Regression called Kernel Regressions and Splines Regressions. As an additional lecture, we discuss a more robust technique on the nonparametric regression. The course requires knowledge of concepts in statistical inference and regression methodology as introduced in the courses Concepts of Probability and Statistics and Linear Models. The statistical software used is mainly R. Thus, ideally students should also have followed the course Programming in R . At the end of the course, the student should be able to decide on the appropriate rank test to be used for a range of real life examples, to be able execute the test and to interpret the results. For the topics discussed relating to nonparametric regression, the students should be able to choose the appropratie nonparametric regression techniques, depending on the characteristics of a real life dataset.
|
|
|
|
|
|
|
Lecture ✔
|
|
|
Project ✔
|
|
|
Self-study assignment ✔
|
|
|
|
|
|
Homework ✔
|
|
|
Paper ✔
|
|
|
Presentation ✔
|
|
|
|
Period 2 Credits 4,00
Evaluation method | |
|
Written evaluaton during teaching periode | 10 % |
|
|
|
|
|
|
Off campus online evaluation/exam | ✔ |
|
For the full evaluation/exam | ✔ |
|
|
|
Second examination period
Evaluation second examination opportunity different from first examination opprt | |
|
|
 
|
Compulsory course material |
|
Nonparametrics, Statistical Methods based on Ranks., E.L. Lehmann and H.J.M. D'Abrera
Applied Nonparametric Regression, Wolfgang Hardle.
Handout by instructors. |
|
|
|
|
|
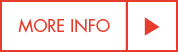 | 1st year Master Bioinformatics - distance learning | Optional | 108 | 4,0 | 108 | 4,0 | Yes | Yes | Numerical |  |
1st year Master Data Science - distance learning | Optional | 108 | 4,0 | 108 | 4,0 | Yes | Yes | Numerical |  |
1st year Master Quantitative Epidemiology - distance learning | Optional | 108 | 4,0 | 108 | 4,0 | Yes | Yes | Numerical |  |
|
| Learning outcomes |
- EC
| The student can handle scientific quantitative research questions, independently, effectively, creatively, and correctly using state-of-the-art design and analysis methodology and software. | | - DC
| ... correctly using state-of-the-art analysis methodology. | | - DC
| ... correctly using state-of-the-art software. | - EC
| The student is capable of acquiring new knowledge. | - EC
| The student is able to efficiently acquire, store and process data. | - EC
| The student can critically appraise methodology and challenge proposals for and reported results of data analysis. | - EC
| The student is an effective written and oral communicator, both within their own field as well as across disciplines. | - EC
| The student can put research and consulting aspects of one or more statistical fields into practice. | | - DC
| The student can put the consulting aspects of one or more statistical fields into practice.
| - EC
| The student is able to correctly use the theory, either methodologically or in an application context or both, thus contributing to scientific research within the field of statistical science, data science, or within the field of application. | | - DC
| The student is able to correctly use the theory in an application context, thus contributing to scientific research within the field of application. | | - DC
| The student is able to correctly use the theory in an application context, thus contributing to scientific research within the field of statistical and data science.
|
|
| EC = learning outcomes DC = partial outcomes BC = evaluation criteria |
|
The student should be familiar with basic concepts of statistics, such as: type I and type II error, hypothesis testing, and basic linear regression methodology. R software will be used for this course.
|
|
|
We will discuss rank test statistics for comparing two independent groups, two dependent groups, and the comparison of more than two independent and dependent groups. In the nonparametric regression part, we will start with Kernel Density functions, and then we introduce two basic approaches on Nonparametric Regression called Kernel Regressions and Splines Regressions. As an additional lecture, we discuss a more robust technique on the nonparametric regression. The course requires knowledge of concepts in statistical inference and regression methodology as introduced in the courses Concepts of Probability and Statistics and Linear Models. The statistical software used is mainly R. Thus, ideally students should also have followed the course Programming in R . At the end of the course, the student should be able to decide on the appropriate rank test to be used for a range of real life examples, to be able execute the test and to interpret the results. For the topics discussed relating to nonparametric regression, the students should be able to choose the appropratie nonparametric regression techniques, depending on the characteristics of a real life dataset.
|
|
|
|
|
|
|
Lecture ✔
|
|
|
Project ✔
|
|
|
Self-study assignment ✔
|
|
|
|
|
|
Homework ✔
|
|
|
Paper ✔
|
|
|
Presentation ✔
|
|
|
|
Period 2 Credits 4,00
Evaluation method | |
|
Written evaluaton during teaching periode | 10 % |
|
|
|
|
|
|
|
Off campus online evaluation/exam | ✔ |
|
For the full evaluation/exam | ✔ |
|
|
|
Second examination period
Evaluation second examination opportunity different from first examination opprt | |
|
|
 
|
Compulsory course material |
|
Nonparametrics, Statistical Methods based on Ranks., E.L. Lehmann and H.J.M. D'Abrera
Applied Nonparametric Regression, Wolfgang Hardle.
Handout by instructors. |
|
|
|
|
|
1 Education, Examination and Legal Position Regulations art.12.2, section 2. |
2 Education, Examination and Legal Position Regulations art.16.9, section 2. |
3 Education, Examination and Legal Position Regulations art.15.1, section 3.
|
Legend |
SBU : course load | SP : ECTS | N : Dutch | E : English |
|