Language of instruction : English |
Exam contract: not possible |
Sequentiality
|
|
No sequentiality
|
| Degree programme | | Study hours | Credits | P1 SBU | P1 SP | 2nd Chance Exam1 | Tolerance2 | Final grade3 | |
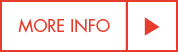 | 1st year Master Bioinformatics | Compulsory | 135 | 5,0 | 135 | 5,0 | Yes | No | Numerical |  |
1st year Master Bioinformatics - icp | Compulsory | 135 | 5,0 | 135 | 5,0 | Yes | No | Numerical |  |
1st year Master Biostatistics | Compulsory | 135 | 5,0 | 135 | 5,0 | Yes | No | Numerical |  |
1st year Master Biostatistics - icp | Compulsory | 135 | 5,0 | 135 | 5,0 | Yes | No | Numerical |  |
1st year Master Data Science | Compulsory | 135 | 5,0 | 135 | 5,0 | Yes | No | Numerical |  |
1st year Quantitative Epidemiology | Compulsory | 135 | 5,0 | 135 | 5,0 | Yes | No | Numerical |  |
1st year Master Quantitative Epidemiology - icp | Compulsory | 135 | 5,0 | 135 | 5,0 | Yes | No | Numerical |  |
|
| Learning outcomes |
- EC
| The student can handle scientific quantitative research questions, independently, effectively, creatively, and correctly using state-of-the-art design and analysis methodology and software. | | - DC
| ... correctly using state-of-the-art analysis methodology. | | - DC
| ... correctly using state-of-the-art software. | - EC
| The student is capable of acquiring new knowledge. | - EC
| The student routinely monitors his/her own learning process and adjusts and improves it accordingly. | - EC
| The student can critically appraise methodology and challenge proposals for and reported results of data analysis. | - EC
| The student has the habit to assess data quality and integrity. | - EC
| The student knows the ethical, moral, legal, policy making, and privacy context of statistics and data science, and always acts accordingly. | | - DC
| The student can explain basic principles regarding ethics and integrity in general. |
|
| EC = learning outcomes DC = partial outcomes BC = evaluation criteria |
|
Knowledge of basic concepts from probability and statistics are required, as well as familiarity with matrix algebra and basic R programming and reporting skills.
|
|
|
This course introduces the student to simple and multiple linear regression models, including analysis of variance. The course starts with an introduction to statistical modelling and then moves to the linear model. The following topics are covered: parameter estimation, statistical inference on the parameters, prediction, model selection and model assessment. The course also focuses on the interpretation of the models and their parameters and the correct use of the models and methods. The student will also learn to perform data analyses with linear models in statistical software (R / SAS) and to correctly report the results of the data analysis.
|
|
|
|
|
|
|
Collective feedback moment ✔
|
|
|
Flipped Classroom ✔
|
|
|
Lecture ✔
|
|
|
Q&A ✔
|
|
|
Self-study assignment ✔
|
|
|
|
|
|
Homework ✔
|
|
|
|
Period 1 Credits 5,00
Evaluation method | |
|
Written evaluaton during teaching periode | 25 % |
|
Transfer of partial marks within the academic year | ✔ |
|
|
|
|
|
|
|
Written exam | 75 % |
|
Transfer of partial marks within the academic year | ✔ |
|
|
|
|
|
|
|
Use of study material during evaluation | ✔ |
|
Explanation (English) | For the final written exam students can use their own hand calculator, lecture notes, own notes, hand-book, statistical tables. |
|
|
|
Evaluation conditions (participation and/or pass) | ✔ |
|
Conditions | To get a pass mark (>9/20) for this course the student must pass for both the homework assignments (average score) and for the final written exam. |
|
|
|
Consequences | If the student did not pass for both the homework assignments and for the final written exam, the total score will be the minimum between: - 9 - the sum of all evaluations (homework assignments and written exam) of the course. |
|
|
|
Second examination period
Evaluation second examination opportunity different from first examination opprt | |
|
|
|
Compulsory course material |
|
All course material (course notes, handouts, video lectures and exercises) will be available on Blackboard |
|
|
|
|
|
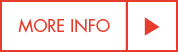 | Master of Teaching in Sciences and Technology - Engineering and Technology choice for subject didactics math | Optional | 135 | 5,0 | 135 | 5,0 | Yes | Yes | Numerical |  |
Exchange Programme Statistics | Optional | 135 | 5,0 | 135 | 5,0 | Yes | Yes | Numerical |  |
|
| Learning outcomes |
- EC
| WET 1. The newly graduated student has advanced knowledge, insight, skills and attitudes in the disciplines relevant to his/her specific subject didactics and is able to communicate these appropriately to his/her stakeholders. |
|
| EC = learning outcomes DC = partial outcomes BC = evaluation criteria |
|
Knowledge of basic concepts from probability and statistics are required, as well as familiarity with matrix algebra and basic R programming and reporting skills.
|
|
|
This course introduces the student to simple and multiple linear regression models, including analysis of variance. The course starts with an introduction to statistical modelling and then moves to the linear model. The following topics are covered: parameter estimation, statistical inference on the parameters, prediction, model selection and model assessment. The course also focuses on the interpretation of the models and their parameters and the correct use of the models and methods. The student will also learn to perform data analyses with linear models in statistical software (R / SAS) and to correctly report the results of the data analysis.
|
|
|
|
|
|
|
Collective feedback moment ✔
|
|
|
Flipped Classroom ✔
|
|
|
Lecture ✔
|
|
|
Q&A ✔
|
|
|
Self-study assignment ✔
|
|
|
|
|
|
Homework ✔
|
|
|
|
Period 1 Credits 5,00
Evaluation method | |
|
Written evaluaton during teaching periode | 25 % |
|
Transfer of partial marks within the academic year | ✔ |
|
|
|
|
|
|
|
Written exam | 75 % |
|
Transfer of partial marks within the academic year | ✔ |
|
|
|
|
|
|
|
Use of study material during evaluation | ✔ |
|
Explanation (English) | For the final written exam students can use their own hand calculator, lecture notes, own notes, hand-book, statistical tables. |
|
|
|
Evaluation conditions (participation and/or pass) | ✔ |
|
Conditions | To get a pass mark (>9/20) for this course the student must pass for both the homework assignments (average score) and for the final written exam. |
|
|
|
Consequences | If the student did not pass for both the homework assignments and for the final written exam, the total score will be the minimum between: - 9 - the sum of all evaluations (homework assignments and written exam) of the course. |
|
|
|
Second examination period
Evaluation second examination opportunity different from first examination opprt | |
|
|
|
Compulsory course material |
|
All course material (course notes, handouts, video lectures and exercises) will be available on Blackboard |
|
|
|
|
|
1 Education, Examination and Legal Position Regulations art.12.2, section 2. |
2 Education, Examination and Legal Position Regulations art.16.9, section 2. |
3 Education, Examination and Legal Position Regulations art.15.1, section 3.
|
Legend |
SBU : course load | SP : ECTS | N : Dutch | E : English |
|