Language of instruction : English |
Exam contract: not possible |
Sequentiality
|
|
Mandatory sequentiality bound on the level of programme components
|
|
|
|
Following programme components must have been included in your study programme in a previous education period
|
|
|
Linear Models (3560)
|
5.0 stptn |
|
|
| Degree programme | | Study hours | Credits | P2 SBU | P2 SP | 2nd Chance Exam1 | Tolerance2 | Final grade3 | |
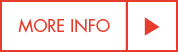 | 1st year Master Bioinformatics | Compulsory | 162 | 6,0 | 162 | 6,0 | Yes | No | Numerical |  |
1st year Master Bioinformatics - icp | Compulsory | 162 | 6,0 | 162 | 6,0 | Yes | No | Numerical |  |
1st year Master Biostatistics | Compulsory | 162 | 6,0 | 162 | 6,0 | Yes | No | Numerical |  |
1st year Master Biostatistics - icp | Compulsory | 162 | 6,0 | 162 | 6,0 | Yes | No | Numerical |  |
1st year Quantitative Epidemiology | Compulsory | 162 | 6,0 | 162 | 6,0 | Yes | No | Numerical |  |
1st year Master Quantitative Epidemiology - icp | Compulsory | 162 | 6,0 | 162 | 6,0 | Yes | No | Numerical |  |
|
| Learning outcomes |
- EC
| The student can handle scientific quantitative research questions, independently, effectively, creatively, and correctly using state-of-the-art design and analysis methodology and software. | | - DC
| ... correctly using state-of-the-art design methodology. | | - DC
| ... correctly using state-of-the-art analysis methodology. | | - DC
| ... correctly using state-of-the-art software. | - EC
| The student is capable of acquiring new knowledge. | - EC
| The student can work in a multidisciplinary, intercultural, and international team. | - EC
| The student knows the international nature of the field of statistical science and data science. |
|
| EC = learning outcomes DC = partial outcomes BC = evaluation criteria |
|
At the end of this course, the student should have a profound knowledge of generalized linear models and basic knowledge of some extensions, including
Part I
- Standard descriptive and inferential methods for multiway contingency t ables (odds ratios, conditional independence, Cochran-Mantel-Haenszel procedures,...)
- Components of a generalized linear model (GLM)
- GLM for binary data: logistic regression
- Building and applying logistic regression models
- Overdispersion and quasi-likelihood
- Conditional logistic regression and exact distributions
Part II
- Extensions to multinomial responses (baseline category, cumulative link, partial odds ratio,...)
- Extensions to clustered binary (GEE, random effects)
- Extensions to clustered & multinomial data
- Loglinear models
- Models for matched pairs
The student should be able to apply such models and methods using appropriate software (SAS, R).
|
|
|
|
|
|
|
Lecture ✔
|
|
|
Self-study assignment ✔
|
|
|
|
Period 2 Credits 6,00
Evaluation method | |
|
Written exam | 100 % |
|
|
Multiple-choice questions | ✔ |
|
|
|
|
|
|
|
Use of study material during evaluation | ✔ |
|
Explanation (English) | Copy slides, text books, notes, copies |
|
|
|
Second examination period
Evaluation second examination opportunity different from first examination opprt | |
|
|
 
|
Compulsory textbooks (bookshop) |
|
Categorical Data Analysis,Agresti, Alan,3rd edition,Wiley,9780470463635 |
|
|
|
|
|
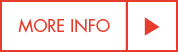 | 1st year Master Data Science | Compulsory | 81 | 3,0 | 81 | 3,0 | Yes | Yes | Numerical |  |
|
| Learning outcomes |
- EC
| The student can handle scientific quantitative research questions, independently, effectively, creatively, and correctly using state-of-the-art design and analysis methodology and software. | | - DC
| ... correctly using state-of-the-art analysis methodology. | | - DC
| ... correctly using state-of-the-art design methodology. | | - DC
| ... correctly using state-of-the-art software. | - EC
| The student is capable of acquiring new knowledge. | - EC
| The student can work in a multidisciplinary, intercultural, and international team. | - EC
| The student knows the international nature of the field of statistical science and data science. |
|
| EC = learning outcomes DC = partial outcomes BC = evaluation criteria |
|
At the end of this course, the student should have a profound knowledge of generalized linear models and basic knowledge of some extensions, including
- Standard descriptive and inferential methods for multiway contingency t ables (odds ratios, conditional independence, Cochran-Mantel-Haenszel procedures,...)
- Components of a generalized linear model (GLM)
- GLM for binary data: logistic regression
- Building and applying logistic regression models
- Overdispersion and quasi-likelihood
- Conditional logistic regression and exact distributions
The student should be able to apply such models and methods using appropriate software (SAS, R).
|
|
|
|
|
|
|
Lecture ✔
|
|
|
Self-study assignment ✔
|
|
|
|
Period 2 Credits 3,00
Evaluation method | |
|
Written exam | 100 % |
|
|
Multiple-choice questions | ✔ |
|
|
|
|
|
|
|
Use of study material during evaluation | ✔ |
|
Explanation (English) | Copy slides, text books, notes, copies |
|
|
|
Second examination period
Evaluation second examination opportunity different from first examination opprt | |
|
|
 
|
Compulsory textbooks (bookshop) |
|
Categorical Data Analysis,Agresti, Alan,3rd edition,Wiley,9780470463635 |
|
|
|
|
|
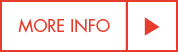 | 1st Master of Business and Information Systems Engineering | Optional | 162 | 6,0 | 162 | 6,0 | Yes | Yes | Numerical |  |
2nd Master of Business and Information Systems Engineering | Optional | 162 | 6,0 | 162 | 6,0 | Yes | Yes | Numerical |  |
Exchange Programme Statistics | Optional | 162 | 6,0 | 162 | 6,0 | Yes | Yes | Numerical |  |
|
| Learning outcomes |
- EC
| The holder of the degree communicates clearly and correctly in writing and orally, in a business and academic context, if necessary supplemented with visual support. (Communication) | - EC
| The holder of the degree shows autonomy in implementing scientific research methods. (Research skills) | - EC
| The holder of the degree shows autonomy in analysing, interpreting, evaluating and reporting research results. (Research skills) |
|
| EC = learning outcomes DC = partial outcomes BC = evaluation criteria |
|
At the end of this course, the student should have a profound knowledge of generalized linear models and basic knowledge of some extensions, including
Part I
- Standard descriptive and inferential methods for multiway contingency t ables (odds ratios, conditional independence, Cochran-Mantel-Haenszel procedures,...)
- Components of a generalized linear model (GLM)
- GLM for binary data: logistic regression
- Building and applying logistic regression models
- Overdispersion and quasi-likelihood
- Conditional logistic regression and exact distributions
Part II
- Extensions to multinomial responses (baseline category, cumulative link, partial odds ratio,...)
- Extensions to clustered binary (GEE, random effects)
- Extensions to clustered & multinomial data
- Loglinear models
- Models for matched pairs
The student should be able to apply such models and methods using appropriate software (SAS, R).
|
|
|
|
|
|
|
Lecture ✔
|
|
|
Self-study assignment ✔
|
|
|
|
Period 2 Credits 6,00
Evaluation method | |
|
Written exam | 100 % |
|
|
Multiple-choice questions | ✔ |
|
|
|
|
|
|
|
Use of study material during evaluation | ✔ |
|
Explanation (English) | Copy slides, text books, notes, copies |
|
|
|
Second examination period
Evaluation second examination opportunity different from first examination opprt | |
|
|
 
|
Compulsory textbooks (bookshop) |
|
Categorical Data Analysis,Agresti, Alan,3rd edition,Wiley,9780470463635 |
|
|
|
|
|
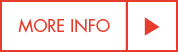 | Exchange Programme Mathematics | Optional | 162 | 6,0 | 162 | 6,0 | Yes | Yes | Numerical |  |
|
|
|
At the end of this course, the student should have a profound knowledge of generalized linear models and basic knowledge of some extensions, including
Part I
- Standard descriptive and inferential methods for multiway contingency t ables (odds ratios, conditional independence, Cochran-Mantel-Haenszel procedures,...)
- Components of a generalized linear model (GLM)
- GLM for binary data: logistic regression
- Building and applying logistic regression models
- Overdispersion and quasi-likelihood
- Conditional logistic regression and exact distributions
Part II
- Extensions to multinomial responses (baseline category, cumulative link, partial odds ratio,...)
- Extensions to clustered binary (GEE, random effects)
- Extensions to clustered & multinomial data
- Loglinear models
- Models for matched pairs
The student should be able to apply such models and methods using appropriate software (SAS, R).
|
|
|
|
|
|
|
Lecture ✔
|
|
|
Self-study assignment ✔
|
|
|
|
Period 2 Credits 6,00
Evaluation method | |
|
Written exam | 100 % |
|
|
Multiple-choice questions | ✔ |
|
|
|
|
|
|
|
Use of study material during evaluation | ✔ |
|
Explanation (English) | Copy slides, text books, notes, copies |
|
|
|
Second examination period
Evaluation second examination opportunity different from first examination opprt | |
|
|
 
|
Compulsory textbooks (bookshop) |
|
Categorical Data Analysis,Agresti, Alan,3rd edition,Wiley,9780470463635 |
|
|
|
|
|
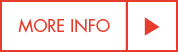 | Master of Teaching in Sciences and Technology - Engineering and Technology choice for subject didactics math | Optional | 162 | 6,0 | 162 | 6,0 | Yes | Yes | Numerical |  |
|
| Learning outcomes |
- EC
| WET 2. The newly graduated student can independently set up and conduct research relevant to his field, consisting of a critical literature study, formulating a research question and hypothesis, selecting and optimizing suitable methods and techniques, critically analyzing and interpreting the results, formulating conclusions and reporting of the findings. |
|
| EC = learning outcomes DC = partial outcomes BC = evaluation criteria |
|
At the end of this course, the student should have a profound knowledge of generalized linear models and basic knowledge of some extensions, including
Part I
- Standard descriptive and inferential methods for multiway contingency t ables (odds ratios, conditional independence, Cochran-Mantel-Haenszel procedures,...)
- Components of a generalized linear model (GLM)
- GLM for binary data: logistic regression
- Building and applying logistic regression models
- Overdispersion and quasi-likelihood
- Conditional logistic regression and exact distributions
Part II
- Extensions to multinomial responses (baseline category, cumulative link, partial odds ratio,...)
- Extensions to clustered binary (GEE, random effects)
- Extensions to clustered & multinomial data
- Loglinear models
- Models for matched pairs
The student should be able to apply such models and methods using appropriate software (SAS, R).
|
|
|
|
|
|
|
Lecture ✔
|
|
|
Self-study assignment ✔
|
|
|
|
Period 2 Credits 6,00
Evaluation method | |
|
Written exam | 100 % |
|
|
Multiple-choice questions | ✔ |
|
|
|
|
|
|
|
Use of study material during evaluation | ✔ |
|
Explanation (English) | Copy slides, text books, notes, copies |
|
|
|
Second examination period
Evaluation second examination opportunity different from first examination opprt | |
|
|
 
|
Compulsory textbooks (bookshop) |
|
Categorical Data Analysis,Agresti, Alan,3rd edition,Wiley,9780470463635 |
|
|
|
|
|
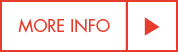 | Exchange Programme Statistics | Optional | 81 | 3,0 | 81 | 3,0 | Yes | Yes | Numerical |  |
|
|
|
At the end of this course, the student should have a profound knowledge of generalized linear models and basic knowledge of some extensions, including
- Standard descriptive and inferential methods for multiway contingency t ables (odds ratios, conditional independence, Cochran-Mantel-Haenszel procedures,...)
- Components of a generalized linear model (GLM)
- GLM for binary data: logistic regression
- Building and applying logistic regression models
- Overdispersion and quasi-likelihood
- Conditional logistic regression and exact distributions
The student should be able to apply such models and methods using appropriate software (SAS, R).
|
|
|
|
|
|
|
Lecture ✔
|
|
|
Self-study assignment ✔
|
|
|
|
Period 2 Credits 3,00
Evaluation method | |
|
Written exam | 100 % |
|
|
Multiple-choice questions | ✔ |
|
|
|
|
|
|
|
Use of study material during evaluation | ✔ |
|
Explanation (English) | Copy slides, text books, notes, copies |
|
|
|
Second examination period
Evaluation second examination opportunity different from first examination opprt | |
|
|
 
|
Compulsory textbooks (bookshop) |
|
Categorical Data Analysis,Agresti, Alan,3rd edition,Wiley,9780470463635 |
|
|
|
|
|
1 Education, Examination and Legal Position Regulations art.12.2, section 2. |
2 Education, Examination and Legal Position Regulations art.16.9, section 2. |
3 Education, Examination and Legal Position Regulations art.15.1, section 3.
|
Legend |
SBU : course load | SP : ECTS | N : Dutch | E : English |
|