Language of instruction : English |
Exam contract: not possible |
Sequentiality
|
|
Mandatory sequentiality bound on the level of programme components
|
|
|
|
Following programme components must have been included in your study programme in a previous education period
|
|
|
Generalized Linear Models (3563)
|
6.0 stptn |
|
|
| Degree programme | | Study hours | Credits | P1 SBU | P1 SP | 2nd Chance Exam1 | Tolerance2 | Final grade3 | |
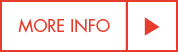 | 2nd year Master Bioinformatics | Optional | 81 | 3,0 | 81 | 3,0 | Yes | Yes | Numerical |  |
2nd year Master Biostatistics | Optional | 81 | 3,0 | 81 | 3,0 | Yes | Yes | Numerical |  |
2nd year Master Data Science | Optional | 81 | 3,0 | 81 | 3,0 | Yes | Yes | Numerical |  |
2nd year Master Quantitative Epidemiology | Optional | 81 | 3,0 | 81 | 3,0 | Yes | Yes | Numerical |  |
2nd year Master Quantitative Epidemiology - icp | Optional | 81 | 3,0 | 81 | 3,0 | Yes | Yes | Numerical |  |
Exchange Programme Statistics | Optional | 81 | 3,0 | 81 | 3,0 | Yes | Yes | Numerical |  |
|
| Learning outcomes |
- EC
| The student can handle scientific quantitative research questions, independently, effectively, creatively, and correctly using state-of-the-art design and analysis methodology and software. | | - DC
| ... correctly using state-of-the-art analysis methodology. | | - DC
| ... correctly using state-of-the-art software. | - EC
| The student is capable of acquiring new knowledge. | - EC
| The student can work in a multidisciplinary, intercultural, and international team. | - EC
| The student is an effective written and oral communicator, both within their own field as well as across disciplines. | | - DC
| The student is an effective writer in their own field. | | - DC
| The student is an effective writer, both within their own field as well as across disciplines. |
|
| EC = learning outcomes DC = partial outcomes BC = evaluation criteria |
|
The student should be familiar with statistical inference and statistical models.
The student should be familiar with programming in R.
|
|
|
Introduction to the different steps (and their integration) of microbial risk assessment.
Statistical, mathematical and simulation methodology for:
- models for quantitative risk assessment
- models for exposure assessment (concentration distribution, limit of detection, distribution of number of organisms)
- models for the quantification of consumption data and dealing with correlated inputs
- mechanistic and empirical dose-response models, infection versus illness, model averaging.
|
|
|
Period 1 Credits 3,00
Evaluation method | |
|
Written evaluaton during teaching periode | 50 % |
|
Transfer of partial marks within the academic year | ✔ |
|
|
|
|
|
|
|
|
Use of study material during evaluation | ✔ |
|
Explanation (English) | Slides, course notes |
|
|
|
Second examination period
Evaluation second examination opportunity different from first examination opprt | |
|
Explanation (English) | Score for project is carried over to the retake exam. |
|
|
|
|
|
Compulsory course material |
|
Lecture notes will be made available via blackboard.
The R software will be used in this course. |
|
|
Recommended reading |
|
Quantitative Microbial Risk Assessment,Charles N. Haas; Joan B. Rose; Charles P. Gerba,Wiley,9780471183976,Nu reeds beschikbaar als e-book @UHasselt: http://bib-proxy.uhasselt.be/login?url=https://ebookcentral.proquest.com/lib/ubhasselt/detail.action?docID=1706900 |
|
|
|
|
|
1 Education, Examination and Legal Position Regulations art.12.2, section 2. |
2 Education, Examination and Legal Position Regulations art.16.9, section 2. |
3 Education, Examination and Legal Position Regulations art.15.1, section 3.
|
Legend |
SBU : course load | SP : ECTS | N : Dutch | E : English |
|