Language of instruction : English |
Exam contract: not possible |
Sequentiality
|
|
No sequentiality
|
| Degree programme | | Study hours | Credits | P1 SBU | P1 SP | 2nd Chance Exam1 | Tolerance2 | Final grade3 | |
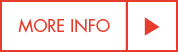 | 1st year Master Bioinformatics - distance learning | Compulsory | 135 | 5,0 | 135 | 5,0 | Yes | No | Numerical |  |
1st year Master Biostatistics - distance learning | Compulsory | 135 | 5,0 | 135 | 5,0 | Yes | No | Numerical |  |
1st year Master Data Science - distance learning | Compulsory | 135 | 5,0 | 135 | 5,0 | Yes | No | Numerical |  |
1st year Master Quantitative Epidemiology - distance learning | Compulsory | 135 | 5,0 | 135 | 5,0 | Yes | No | Numerical |  |
|
| Learning outcomes |
- EC
| The student is capable of acquiring new knowledge. | - EC
| The student is able to correctly use the theory, either methodologically or in an application context or both, thus contributing to scientific research within the field of statistical science, data science, or within the field of application. | | - DC
| The student is able to correctly use the theory methodologically, thus contributing to scientific research within the field of statistical and data science.
|
|
| EC = learning outcomes DC = partial outcomes BC = evaluation criteria |
|
The student has a strong knowledge of mathematics.
In the following mathematical topics, the student has a ready knowledge of the calculation techniques:
- Set theory
- Functions (univariate and multivariate)
- Limits and Infinite Sequences
- Sums and Series
- Derivatives (univariate and multivariate) and optimization problems
- Integrals ((In-)Definite and improper integrals, Gamma and Beta functions).
|
|
|
In this course, we deal with the following topics:
- Descriptive statistics:
- different types of variables
- measures of centrality
- measures of variability
- measures of relative standing
- graphical methods to present data
- Basic probability theory:
- sample space, events, probability, combinatorics
- Law of total probability, Bayes rule
- stochastic variables, (joint, conditional) distributions, (conditional) expectations
- transformation of distributions
- Law of large numbers, Central limit theorem
- generating samples from a population
- Statistical inference:
- Confidence intervals: CI for mean(s), CI for proportion(s), CI for variance(s)
- hypothesis testing: null-hypothesis, alternative hypothesis, test-statistic, critical value, p-value
- hypothesis for mean, proportion and variance
- comparing means, proportions, variances
- Introduction to estimation methods: maximum likelihood, methods of moments, least squares method
|
|
|
|
|
|
|
Collective feedback moment ✔
|
|
|
Distance learning ✔
|
|
|
|
|
|
Exercises ✔
|
|
|
|
Period 1 Credits 5,00
Evaluation method | |
|
Written evaluaton during teaching periode | 10 % |
|
|
|
|
|
Off campus online evaluation/exam | ✔ |
|
For the full evaluation/exam | ✔ |
|
|
|
Second examination period
Evaluation second examination opportunity different from first examination opprt | |
|
|
 
|
Compulsory textbooks (bookshop) |
|
Mathematical Statistics and Data Analysis,John A. Rice,Third Edition,Cengage,9780495118688 |
|
 
|
Compulsory course material |
|
Lecture notes for the lectures will be provided by the lecturer through the electronic platform.
R and R-studio will be used as softwares in this course. |
|
|
|
|
|
1 Education, Examination and Legal Position Regulations art.12.2, section 2. |
2 Education, Examination and Legal Position Regulations art.16.9, section 2. |
3 Education, Examination and Legal Position Regulations art.15.1, section 3.
|
Legend |
SBU : course load | SP : ECTS | N : Dutch | E : English |
|