Language of instruction : English |
Exam contract: not possible |
Sequentiality
|
|
Mandatory sequentiality bound on the level of programme components
|
|
|
|
Following programme components must have been included in your study programme in a previous education period
|
|
|
Concepts of Probability and Statistics DL (3220)
|
5.0 stptn |
|
|
Generalized Linear Models DL (3580)
|
6.0 stptn |
|
|
Longitudinal Data Analysis DL (3784)
|
6.0 stptn |
|
|
Medical and Molecular Biology DL (3581)
|
4.0 stptn |
|
|
Principles of Statistical Inference DL (3787)
|
3.0 stptn |
|
|
Survival Data Analysis DL (3632)
|
3.0 stptn |
|
|
| Degree programme | | Study hours | Credits | P2 SBU | P2 SP | 2nd Chance Exam1 | Tolerance2 | Final grade3 | |
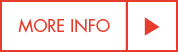 | second year Master Biostatistics - distance learning | Compulsory | 135 | 5,0 | 135 | 5,0 | Yes | No | Numerical |  |
|
| Learning outcomes |
- EC
| The student can handle scientific quantitative research questions, independently, effectively, creatively, and correctly using state-of-the-art design and analysis methodology and software. | - EC
| The student is capable of acquiring new knowledge. | - EC
| The student knows the international nature of the field of statistical science and data science. | - EC
| The student knows the ethical, moral, legal, policy making, and privacy context of statistics and data science, and always acts accordingly. | - EC
| The student is an effective written and oral communicator, both within their own field as well as across disciplines. | | - DC
| The student is an effective oral communicator in their own field. | | - DC
| The student is an effective oral communicator, both within their own field as well as across disciplines. | - EC
| The student knows the relevant stakeholders and understands the need for assertive and empathic interaction with them. | | - DC
| The student can identify relevant stakeholders and their interests, particularly within the programme specialization. | | - DC
| The student can reflect on the role of the statistician and data scientist in the interaction with the stakeholders. | | - DC
| The student can, when building an argumentation, consider different perspectives and interests. | | - DC
| The student can explain the consequences of his/her work for relevant stakeholders. | - EC
| The student is able to correctly use the theory, either methodologically or in an application context or both, thus contributing to scientific research within the field of statistical science, data science, or within the field of application. | | - DC
| The student is able to correctly use the theory in an application context, thus contributing to scientific research within the field of statistical and data science.
| | - DC
| The student is able to correctly use the theory in an application context, thus contributing to scientific research within the field of application. |
|
| EC = learning outcomes DC = partial outcomes BC = evaluation criteria |
|
The student should be familiar with statistical inference, statistical (generalized linear, mixed effects) models, basic methods of survival analysis.
|
|
|
Topics: Phase I trial designs; Phase II trials designs; Phase III Trial objectives (measures of treatment effect; statistical inference: hypotheses, significance and power; testing for difference or benefit; testing for equivalence or non-inferiority); Treatments (controls (placebo, active control); experimental groups; randomization); Designs (multiple-comparison designs; dose-finding designs; factorial designs; cross-over designs); Patient selection (target population and eligibility criteria; analysis populations; subset analyses; prognostic and predictive factors); Endpoints (follow-up and patient assessments; safety endpoints; efficacy endpoints);Sample size (Type I and Type II errors; sample-size calculations for binary, normal and survival endpoints); Interim analyses (sequential and group-sequential designs; Data Monitoring Committees).
|
|
|
|
|
|
|
Collective feedback moment ✔
|
|
|
Distance learning ✔
|
|
|
|
|
|
Homework ✔
|
|
|
Presentation ✔
|
|
|
|
Period 2 Credits 5,00
Evaluation method | |
|
Written evaluaton during teaching periode | 5 % |
|
Transfer of partial marks within the academic year | ✔ |
|
|
|
|
|
|
Other evaluation method during teaching period | 25 % |
|
Other | Homework projects with inidividual presentations |
|
|
|
Transfer of partial marks within the academic year | ✔ |
|
|
|
|
|
Written exam | 70 % |
|
|
Multiple-choice questions | ✔ |
|
|
|
|
|
Off campus online evaluation/exam | ✔ |
|
For the full evaluation/exam | ✔ |
|
|
|
Additional information | To get the final score, the weighted score is rounded mathematically, unless exam result is less than 50%, in which case the integer part is taken. The maximum final score is 20. To pass the course, the achieved final score has to be at least 10 (i.e., 50%). The quizzes and homework scores are retained when computing the final score after the second chance exam. |
|
Second examination period
Evaluation second examination opportunity different from first examination opprt | |
|
|
 
|
Compulsory course material |
|
Photocopies of relevant book chapters and papers (available in an electronic form on BlackBoard). |
|
 
|
Recommended reading |
|
- Clinical Trials: A Methodologic Perspective, Steven Piantadosi, 2, Wiley, 9780471727811,Available as e-book: https://ebookcentral-proquest-com.bib-proxy.uhasselt.be/lib/ubhasselt/detail.action?docID=5098734&pq-origsite=summon
- Biostatistics in Clinical Trials, Carol K. Redmond; Theodore Colton, Wiley, 9780471822110
- Cancer Clinical Trials: Methods and Practice, Marc E. Buyse; Maurice J. Staquet; Richard J. Sylvester, Oxford University Press, 9780192617651
- Group Sequential Methods with Applications to Clinical Trials, Christopher Jennison; Bruce W. Turnbull, Chapman and Hall/CRC, 9780849303166
- Fundamentals of Clinical Trials, Friedman, L.M.; Furberg, C.D.; DeMets, D.; Reboussin, D.M.; Granger, C.B., 5, Springer, 9783319185385,Available as e-book: https://link-springer-com.bib-proxy.uhasselt.be/book/10.1007%2F978-3-319-18539-2
|
|
 
|
Recommended course material |
|
The use of R software is recommended in this course. |
|
|
|
|
|
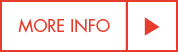 | second year Master Bioinformatics - distance learning | Optional | 135 | 5,0 | 135 | 5,0 | Yes | Yes | Numerical |  |
second year Quantitative Epidemiology - distance learning | Optional | 135 | 5,0 | 135 | 5,0 | Yes | Yes | Numerical |  |
|
| Learning outcomes |
- EC
| The student can handle scientific quantitative research questions, independently, effectively, creatively, and correctly using state-of-the-art design and analysis methodology and software. | - EC
| The student is capable of acquiring new knowledge. | - EC
| The student knows the international nature of the field of statistical science and data science. | - EC
| The student knows the ethical, moral, legal, policy making, and privacy context of statistics and data science, and always acts accordingly. | - EC
| The student is an effective written and oral communicator, both within their own field as well as across disciplines. | | - DC
| The student is an effective oral communicator in their own field. | | - DC
| The student is an effective oral communicator, both within their own field as well as across disciplines. | - EC
| The student knows the relevant stakeholders and understands the need for assertive and empathic interaction with them. | | - DC
| The student can identify relevant stakeholders and their interests, particularly within the programme specialization. | | - DC
| The student can reflect on the role of the statistician and data scientist in the interaction with the stakeholders. | | - DC
| The student can, when building an argumentation, consider different perspectives and interests. | | - DC
| The student can explain the consequences of his/her work for relevant stakeholders. | - EC
| The student is able to correctly use the theory, either methodologically or in an application context or both, thus contributing to scientific research within the field of statistical science, data science, or within the field of application. | | - DC
| The student is able to correctly use the theory in an application context, thus contributing to scientific research within the field of statistical and data science.
| | - DC
| The student is able to correctly use the theory in an application context, thus contributing to scientific research within the field of application. |
|
| EC = learning outcomes DC = partial outcomes BC = evaluation criteria |
|
The student should be familiar with statistical inference, statistical (generalized linear, mixed effects) models, basic methods of survival analysis.
|
|
|
Topics: Phase I trial designs; Phase II trials designs; Phase III Trial objectives (measures of treatment effect; statistical inference: hypotheses, significance and power; testing for difference or benefit; testing for equivalence or non-inferiority); Treatments (controls (placebo, active control); experimental groups; randomization); Designs (multiple-comparison designs; dose-finding designs; factorial designs; cross-over designs); Patient selection (target population and eligibility criteria; analysis populations; subset analyses; prognostic and predictive factors); Endpoints (follow-up and patient assessments; safety endpoints; efficacy endpoints);Sample size (Type I and Type II errors; sample-size calculations for binary, normal and survival endpoints); Interim analyses (sequential and group-sequential designs; Data Monitoring Committees).
|
|
|
|
|
|
|
Collective feedback moment ✔
|
|
|
Distance learning ✔
|
|
|
|
|
|
Homework ✔
|
|
|
Presentation ✔
|
|
|
|
Period 2 Credits 5,00
Evaluation method | |
|
Written evaluaton during teaching periode | 5 % |
|
Transfer of partial marks within the academic year | ✔ |
|
|
|
|
|
|
Other evaluation method during teaching period | 25 % |
|
Other | Homework projects with inidividual presentations |
|
|
|
Transfer of partial marks within the academic year | ✔ |
|
|
|
|
|
Written exam | 70 % |
|
|
Multiple-choice questions | ✔ |
|
|
|
|
|
Off campus online evaluation/exam | ✔ |
|
For the full evaluation/exam | ✔ |
|
|
|
Additional information | To get the final score, the weighted score is rounded mathematically, unless exam result is less than 50%, in which case the integer part is taken. The maximum final score is 20. To pass the course, the achieved final score has to be at least 10 (i.e., 50%). The quizzes and homework scores are retained when computing the final score after the second chance exam. |
|
Second examination period
Evaluation second examination opportunity different from first examination opprt | |
|
|
 
|
Compulsory course material |
|
Photocopies of relevant book chapters and papers (available in an electronic form on BlackBoard). |
|
 
|
Recommended reading |
|
- Clinical Trials: A Methodologic Perspective, Steven Piantadosi, 2, Wiley, 9780471727811,Available as e-book: https://ebookcentral-proquest-com.bib-proxy.uhasselt.be/lib/ubhasselt/detail.action?docID=5098734&pq-origsite=summon
- Biostatistics in Clinical Trials, Carol K. Redmond; Theodore Colton, Wiley, 9780471822110
- Cancer Clinical Trials: Methods and Practice, Marc E. Buyse; Maurice J. Staquet; Richard J. Sylvester, Oxford University Press, 9780192617651
- Group Sequential Methods with Applications to Clinical Trials, Christopher Jennison; Bruce W. Turnbull, Chapman and Hall/CRC, 9780849303166
- Fundamentals of Clinical Trials, Friedman, L.M.; Furberg, C.D.; DeMets, D.; Reboussin, D.M.; Granger, C.B., 5, Springer, 9783319185385,Available as e-book: https://link-springer-com.bib-proxy.uhasselt.be/book/10.1007%2F978-3-319-18539-2
|
|
 
|
Recommended course material |
|
The use of R software is recommended in this course. |
|
|
|
|
|
1 Education, Examination and Legal Position Regulations art.12.2, section 2. |
2 Education, Examination and Legal Position Regulations art.16.9, section 2. |
3 Education, Examination and Legal Position Regulations art.15.1, section 3.
|
Legend |
SBU : course load | SP : ECTS | N : Dutch | E : English |
|