Language of instruction : English |
Exam contract: not possible |
Sequentiality
|
|
Mandatory sequentiality bound on the level of programme components
|
|
|
|
Following programme components must have been included in your study programme in a previous education period
|
|
|
Concepts of Epidemiology (3567)
|
4.0 stptn |
|
|
Concepts of Probability and Statistics (1798)
|
5.0 stptn |
|
|
Data Management (4405)
|
5.0 stptn |
|
|
Generalized Linear Models (3563)
|
6.0 stptn |
|
|
Introduction of Bayesian Inference (3562)
|
4.0 stptn |
|
|
Linear Models (3560)
|
5.0 stptn |
|
|
Medical and Molecular Biology (3564)
|
6.0 stptn |
|
|
Programming in R (4406)
|
3.0 stptn |
|
|
Project: Learning from Data (1113)
|
5.0 stptn |
|
|
Project: Multivariate and Hierarchical Data (3565)
|
8.0 stptn |
|
|
Sampling Theory (1800)
|
5.0 stptn |
|
|
| Degree programme | | Study hours | Credits | P2 SBU | P3 SBU | P3 SP | 2nd Chance Exam1 | Tolerance2 | Final grade3 | |
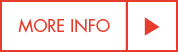 | 2nd year Master Quantitative Epidemiology | Compulsory | 648 | 24,0 | 243 | 405 | 24,0 | Yes | No | Numerical |  |
2nd year Master Quantitative Epidemiology - icp | Compulsory | 648 | 24,0 | 243 | 405 | 24,0 | Yes | No | Numerical |  |
|
| Learning outcomes |
- EC
| The student can handle scientific quantitative research questions, independently, effectively, creatively, and correctly using state-of-the-art design and analysis methodology and software. | | - DC
| ... correctly using state-of-the-art analysis methodology. | | - DC
| ... correctly using state-of-the-art design methodology. | | - DC
| ... correctly using state-of-the-art software. | - EC
| The student is capable of acquiring new knowledge. | - EC
| The student can critically appraise methodology and challenge proposals for and reported results of data analysis. | - EC
| The student has the habit to assess data quality and integrity. | - EC
| The student can work in a multidisciplinary, intercultural, and international team. | - EC
| The student knows the international nature of the field of statistical science and data science. | - EC
| The student knows the societal relevance of statistics and data science. | | - DC
| The student can reflect on and explain the societal relevance of a task, particularly within the programme specialization | | - DC
| The student can reflect on societal tendencies, particularly within the programme specialization. | - EC
| The student knows the ethical, moral, legal, policy making, and privacy context of statistics and data science, and always acts accordingly. | | - DC
| The student can explain basic principles regarding ethics and integrity in general. | | - DC
| The student can apply basic principles regarding ethics and integrity to the fields of statistics and data science. | | - DC
| The student can explain ethical issues and dilemmas within the fields of statistics and data science. | | - DC
| The student acts according to societal and ethical standards in general and particularly within the fields of statistics and data science. | | - DC
| The student respects the privacy of data, people and organizations with whom he/she comes into direct or indirect contact. | - EC
| The student is an effective written and oral communicator, both within their own field as well as across disciplines. | | - DC
| The student is an effective writer in their own field. | | - DC
| The student is an effective writer, both within their own field as well as across disciplines. | | - DC
| The student is an effective oral communicator in their own field. | | - DC
| The student is an effective oral communicator, both within their own field as well as across disciplines. | - EC
| The student knows the relevant stakeholders and understands the need for assertive and empathic interaction with them. | | - DC
| The student can identify relevant stakeholders and their interests, particularly within the programme specialization. | | - DC
| The student can respond to the interests of relevant stakeholders, particularly within the programme specialisation. | | - DC
| The student can reflect on the role of the statistician and data scientist in the interaction with the stakeholders. | | - DC
| The student can, when building an argumentation, consider different perspectives and interests. | | - DC
| The student can explain the consequences of his/her work for relevant stakeholders. | - EC
| The student is able to correctly use the theory, either methodologically or in an application context or both, thus contributing to scientific research within the field of statistical science, data science, or within the field of application. | | - DC
| The student is able to correctly use the theory methodologically, thus contributing to scientific research within the field of statistical and data science.
| | - DC
| The student is able to correctly use the theory methodologically, thus contributing to scientific research within the field of application. | | - DC
| The student is able to correctly use the theory in an application context, thus contributing to scientific research within the field of statistical and data science.
| | - DC
| The student is able to correctly use the theory in an application context, thus contributing to scientific research within the field of application. |
|
| EC = learning outcomes DC = partial outcomes BC = evaluation criteria |
|
Remote or on-site project work: during at least a two-month period, the student works on a real-life research project. The research environment can be an external one, i.e. the student works on a project in collaboration with a a company, research institute, university, governmental institute, .. or an internal one, i.e. the student works on a research project conducted at Hasselt University. Depending on the content of the project and the preferences of the eventual external partner, the student may be required to spend some time at the external partner's premises.
An internal supervisor (Hasselt University or visiting faculty) is always assigned. An external supervisor (responsible for the student at the project place) is assigned in case of an external project.
The project should be representative for the work done by an applied statistician in a real-life environment.
|
|
|
Period 3 Credits 24,00
Evaluation method | |
|
Other exam | 100 % |
|
Other | Students are evaluated based on the thesis text (report), conduct/process and oral defence (oral presentation and replies to questions from the jury) |
|
|
|
|
|
|
Evaluation conditions (participation and/or pass) | ✔ |
|
Conditions | In order to pass the master thesis, the student should pass on each of the evaluation components: the midterm presentation, the report, the conduct/process, the oral defence (presentation and Q&A). The final score is based on a weighted average of the scores on each evaluation component given by the internal promoter (scores all components) and second reader (scores all components except for the conduct). |
|
|
|
Consequences | Students who fail one of the evaluation components of the master thesis (midterm presentation, report, conduct/process, oral defence (presentation and Q&A), cannot pass. |
|
|
|
Additional information | The subject of the master thesis is only valid for one academic year and will hence expire if the student does not pass the master thesis during that academic year (retake exam period included), unless the Examination Board decides otherwise. Students can find more information about the thesis regulations on Blackboard. |
|
Second examination period
Evaluation second examination opportunity different from first examination opprt | |
|
|
 
|
Compulsory course material |
|
Depends on the specific project. |
|
|
|
|
|
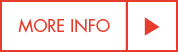 | Exchange Programme Statistics | Optional | 648 | 24,0 | 243 | 405 | 24,0 | Yes | Yes | Numerical |  |
|
|
|
Remote or on-site project work: during at least a two-month period, the student works on a real-life research project. The research environment can be an external one, i.e. the student works on a project in collaboration with a a company, research institute, university, governmental institute, .. or an internal one, i.e. the student works on a research project conducted at Hasselt University. Depending on the content of the project and the preferences of the eventual external partner, the student may be required to spend some time at the external partner's premises.
An internal supervisor (Hasselt University or visiting faculty) is always assigned. An external supervisor (responsible for the student at the project place) is assigned in case of an external project.
The project should be representative for the work done by an applied statistician in a real-life environment.
|
|
|
Period 3 Credits 24,00
Evaluation method | |
|
Other exam | 100 % |
|
Other | master thesis: Students are evaluated based on the thesis text (report), conduct/process and oral defence (oral presentation and replies to questions from the jury) |
|
|
|
|
|
|
Evaluation conditions (participation and/or pass) | ✔ |
|
Conditions | In order to pass the master thesis, the student should pass on each of the evaluation components: the midterm presentation, the report, the conduct/process, the oral defence (presentation and Q&A). The final score is based on a weighted average of the scores on each evaluation component given by the internal promoter (scores all components) and second reader (scores all components except for the conduct). |
|
|
|
Consequences | Students who fail one of the evaluation components of the master thesis (midterm presentation, report, conduct/process, oral defence (presentation and Q&A), cannot pass. |
|
|
|
Additional information | The subject of the master thesis is only valid for one academic year and will hence expire if the student does not pass the master thesis during that academic year (retake exam period included), unless the Examination Board decides otherwise. Students can find more information about the thesis regulations on Blackboard. |
|
Second examination period
Evaluation second examination opportunity different from first examination opprt | |
|
|
 
|
Compulsory course material |
|
Depends on the specific project. |
|
|
|
|
|
1 Education, Examination and Legal Position Regulations art.12.2, section 2. |
2 Education, Examination and Legal Position Regulations art.16.9, section 2. |
3 Education, Examination and Legal Position Regulations art.15.1, section 3.
|
Legend |
SBU : course load | SP : ECTS | N : Dutch | E : English |
|