Language of instruction : English |
| Exam contract: not possible |
Sequentiality
|
|
Mandatory sequentiality bound on the level of programme components
|
|
|
|
Following programme components must have been included in your study programme in a previous education period
|
|
|
Programming in Python (3306)
|
5.0 stptn |
|
|
There is no data for this choice. Change the language, year or choose another item in the dropdown list if it is available.
| Degree programme | | Study hours | Credits | P2 SBU | P2 SP | 2nd Chance Exam1 | Tolerance2 | Final grade3 | |
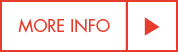 | 1st year Master Data Science | Compulsory | 108 | 4,0 | 108 | 4,0 | Yes | Yes | Numerical |  |
|
| Learning outcomes |
- EC
| The student can work in a multidisciplinary, intercultural, and international team. | | - DC
| The student is able to extract user tasks from domain experts. | - EC
| The student knows the societal relevance of statistics and data science. | | - DC
| The student can reflect on and explain the societal relevance of a task, particularly within the programme specialization | - EC
| The student knows the relevant stakeholders and understands the need for assertive and empathic interaction with them. |
|
| EC = learning outcomes DC = partial outcomes BC = evaluation criteria |
|
As data becomes easier and cheaper to generate, we are moving from a hypothesis-driven to data-driven paradigm in scientific research. As a result, we don't only need to find ways to answer any questions we have, but also to identify interesting questions/hypotheses in that data in the first place. In other words: we need to be able to dig through these large and complex datasets in search for unexpected patterns that - once discovered - can be investigated further using regular statistics and machine learning. Interactive data visualization provides a methodology for just that: to allow the user (be they domain expert or lay user) to find those questions, and to give them deep insight in their data.
Content
- Background and context of data visualization and visual data analysis
- Design as a process: framing the problem, ideation, sketching, design critique, ...
- Programming visualizations: static and dynamic
|
|
|
|
|
|
|
Lecture ✔
|
|
|
Project ✔
|
|
|
Small group session ✔
|
|
|
|
Period 2 Credits 4,00
Evaluation method | |
|
Written evaluaton during teaching periode | 100 % |
|
|
|
|
|
Second examination period
Evaluation second examination opportunity different from first examination opprt | |
|
|
 
|
Recommended reading |
|
- [Visualization Analysis and Design],[Tamara Munzner],[],[A K Peters],[],[Much of the course material is taken from this book]
- [Making Data Visual],[Danyel Fischer & Miriah Meyer],[],[O'Reilly],[],[Very good introductory text]
- [Gamestorming: A Playbook for Innovators, Rulebreakers, and Changemakers],[Dave Gray, Sunni Brown, James Macanufo],[],[O'Reilly],[],[]
|
|
|
|
|
|
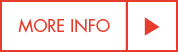 | 1st year Master Bioinformatics | Optional | 108 | 4,0 | 108 | 4,0 | Yes | Yes | Numerical |  |
1st year Master Biostatistics | Optional | 108 | 4,0 | 108 | 4,0 | Yes | Yes | Numerical |  |
1st year Quantitative Epidemiology | Optional | 108 | 4,0 | 108 | 4,0 | Yes | Yes | Numerical |  |
|
| Learning outcomes |
- EC
| The student can work in a multidisciplinary, intercultural, and international team. | | - DC
| The student is able to extract user tasks from domain experts. | - EC
| The student knows the societal relevance of statistics and data science. | | - DC
| The student can reflect on and explain the societal relevance of a task, particularly within the programme specialization |
|
| EC = learning outcomes DC = partial outcomes BC = evaluation criteria |
|
As data becomes easier and cheaper to generate, we are moving from a hypothesis-driven to data-driven paradigm in scientific research. As a result, we don't only need to find ways to answer any questions we have, but also to identify interesting questions/hypotheses in that data in the first place. In other words: we need to be able to dig through these large and complex datasets in search for unexpected patterns that - once discovered - can be investigated further using regular statistics and machine learning. Interactive data visualization provides a methodology for just that: to allow the user (be they domain expert or lay user) to find those questions, and to give them deep insight in their data.
Content
- Background and context of data visualization and visual data analysis
- Design as a process: framing the problem, ideation, sketching, design critique, ...
- Programming visualizations: static and dynamic
|
|
|
|
|
|
|
Lecture ✔
|
|
|
Project ✔
|
|
|
Small group session ✔
|
|
|
|
Period 2 Credits 4,00
Evaluation method | |
|
Written evaluaton during teaching periode | 100 % |
|
|
|
|
|
|
|
Exam method in consultation with the student | 0 % |
|
|
|
|
Second examination period
Evaluation second examination opportunity different from first examination opprt | |
|
|
 
|
Recommended reading |
|
- [Visualization Analysis and Design],[Tamara Munzner],[],[A K Peters],[],[Much of the course material is taken from this book]
- [Making Data Visual],[Danyel Fischer & Miriah Meyer],[],[O'Reilly],[],[Very good introductory text]
- [Gamestorming: A Playbook for Innovators, Rulebreakers, and Changemakers],[Dave Gray, Sunni Brown, James Macanufo],[],[O'Reilly],[],[]
|
|
|
|
|
|
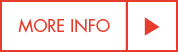 | Exchange Programme Biology | Optional | 108 | 4,0 | 108 | 4,0 | Yes | Yes | Numerical |  |
Exchange Programme Chemistry | Optional | 108 | 4,0 | 108 | 4,0 | Yes | Yes | Numerical |  |
Exchange Programme Physics | Optional | 108 | 4,0 | 108 | 4,0 | Yes | Yes | Numerical |  |
Exchange Programme Computer Science | Optional | 108 | 4,0 | 108 | 4,0 | Yes | Yes | Numerical |  |
Exchange Programme Statistics | Optional | 108 | 4,0 | 108 | 4,0 | Yes | Yes | Numerical |  |
Exchange Programme Mathematics | Optional | 108 | 4,0 | 108 | 4,0 | Yes | Yes | Numerical |  |
|
|
|
As data becomes easier and cheaper to generate, we are moving from a hypothesis-driven to data-driven paradigm in scientific research. As a result, we don't only need to find ways to answer any questions we have, but also to identify interesting questions/hypotheses in that data in the first place. In other words: we need to be able to dig through these large and complex datasets in search for unexpected patterns that - once discovered - can be investigated further using regular statistics and machine learning. Interactive data visualization provides a methodology for just that: to allow the user (be they domain expert or lay user) to find those questions, and to give them deep insight in their data.
Content
- Background and context of data visualization and visual data analysis
- Design as a process: framing the problem, ideation, sketching, design critique, ...
- Programming visualizations: static and dynamic
|
|
|
|
|
|
|
Lecture ✔
|
|
|
Project ✔
|
|
|
Small group session ✔
|
|
|
|
Period 2 Credits 4,00
Evaluation method | |
|
Written evaluaton during teaching periode | 100 % |
|
|
|
|
|
Second examination period
Evaluation second examination opportunity different from first examination opprt | |
|
|
 
|
Recommended reading |
|
- [Visualization Analysis and Design],[Tamara Munzner],[],[A K Peters],[],[Much of the course material is taken from this book]
- [Making Data Visual],[Danyel Fischer & Miriah Meyer],[],[O'Reilly],[],[Very good introductory text]
- [Gamestorming: A Playbook for Innovators, Rulebreakers, and Changemakers],[Dave Gray, Sunni Brown, James Macanufo],[],[O'Reilly],[],[]
|
|
|
|
|
|
1 Education, Examination and Legal Position Regulations art.12.2, section 2. |
2 Education, Examination and Legal Position Regulations art.16.9, section 2. |
3 Education, Examination and Legal Position Regulations art.15.1, section 3.
|
Legend |
SBU : course load | SP : ECTS | N : Dutch | E : English |
|