Language of instruction : English |
| Exam contract: not possible |
Sequentiality
|
|
Mandatory sequentiality bound on the level of programme components
|
|
|
|
Following programme components must have been included in your study programme in a previous education period
|
|
|
Generalized Linear Models (3563)
|
6.0 stptn |
|
|
Project: Multivariate and Hierarchical Data (3565)
|
8.0 stptn |
|
|
There is no data for this choice. Change the language, year or choose another item in the dropdown list if it is available.
| Degree programme | | Study hours | Credits | P1 SBU | P1 SP | 2nd Chance Exam1 | Tolerance2 | Final grade3 | |
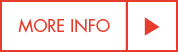 | 2nd year Master Quantitative Epidemiology | Compulsory | 162 | 6,0 | 162 | 6,0 | Yes | No | Numerical |  |
2nd year Master Quantitative Epidemiology - icp | Compulsory | 162 | 6,0 | 162 | 6,0 | Yes | No | Numerical |  |
|
| Learning outcomes |
- EC
| The student is capable of acquiring new knowledge. | - EC
| The student can work in a multidisciplinary, intercultural, and international team. | - EC
| The student is an effective written and oral communicator, both within their own field as well as across disciplines. | | - DC
| The student is an effective writer in their own field. | | - DC
| The student is an effective oral communicator in their own field. | - EC
| The student is able to correctly use the theory, either methodologically or in an application context or both, thus contributing to scientific research within the field of statistical science, data science, or within the field of application. | | - DC
| The student is able to correctly use the theory methodologically, thus contributing to scientific research within the field of statistical and data science.
| | - DC
| The student is able to correctly use the theory methodologically, thus contributing to scientific research within the field of application. | | - DC
| The student is able to correctly use the theory in an application context, thus contributing to scientific research within the field of statistical and data science.
| | - DC
| The student is able to correctly use the theory in an application context, thus contributing to scientific research within the field of application. | | - DC
| The student is able to extract new knowledge and insights from datasets in the application domain. |
|
| EC = learning outcomes DC = partial outcomes BC = evaluation criteria |
|
The student should be familiar with statistical inference, statistical models, survival analysis.
The student should be familiar with programming in R.
|
|
|
Introduction & the basic SIR model (Kermack and McKendrick, 1927), Stochastic models & multiple subpopulations, Metapopulation models, Individual-based models, The analysis of serological data, The analysis of epidemic data, The analysis of surveillance data, Within-host models, Case studies I & II
|
|
|
Period 1 Credits 6,00
Evaluation method | |
|
|
Oral exam | 50 % |
|
Other | Questions about homeworks and project |
|
|
|
|
|
Second examination period
Evaluation second examination opportunity different from first examination opprt | |
|
|
 
|
Compulsory course material |
|
The R software will be used in this course. |
|
 
|
Recommended reading |
|
- [Modeling Infectious Disease Parameters Based on Serological and Social Contact Data: A Modern Statistical Perspective],[Niel Hens; Ziv Shkedy; Marc Aerts; Christel Faes; Pierre Van Damme; Philippe Beutels],[],[Springer New York],[9781461440710],[Available as e-book: https://link.springer.com/book/10.1007%2F978-1-4614-4072-7]
- [Infectious Diseases of Humans: Dynamics and Control],[Roy M. Anderson; Robert M. May],[],[Oxford University Press],[9780198540403],[]
- [Handbook of Infectious Disease Data Analysis],[Leonhard Held, Niel Hens, Philip D O'Neill, Jacco Wallinga],[1st edition],[CRC Press],[9781032087351],[]
- [An Introduction to Infectious Disease Modelling],[Emilia Vynnycky, Richard White],[],[Oxford University Press, USA],[9780198565765],[]
|
|
 
|
Recommended course material |
|
R and Python will be used in this course.
|
|
|
|
|
|
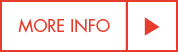 | 2nd year Master Bioinformatics | Optional | 162 | 6,0 | 162 | 6,0 | Yes | Yes | Numerical |  |
2nd year Master Biostatistics | Optional | 162 | 6,0 | 162 | 6,0 | Yes | Yes | Numerical |  |
2nd year Master Data Science | Optional | 162 | 6,0 | 162 | 6,0 | Yes | Yes | Numerical |  |
Exchange Programme Statistics | Optional | 162 | 6,0 | 162 | 6,0 | Yes | Yes | Numerical |  |
|
| Learning outcomes |
- EC
| The student is capable of acquiring new knowledge. | - EC
| The student can work in a multidisciplinary, intercultural, and international team. | - EC
| The student is an effective written and oral communicator, both within their own field as well as across disciplines. | | - DC
| The student is an effective writer in their own field. | | - DC
| The student is an effective oral communicator in their own field. | - EC
| The student is able to correctly use the theory, either methodologically or in an application context or both, thus contributing to scientific research within the field of statistical science, data science, or within the field of application. | | - DC
| The student is able to correctly use the theory methodologically, thus contributing to scientific research within the field of statistical and data science.
| | - DC
| The student is able to correctly use the theory methodologically, thus contributing to scientific research within the field of application. | | - DC
| The student is able to correctly use the theory in an application context, thus contributing to scientific research within the field of statistical and data science.
| | - DC
| The student is able to correctly use the theory in an application context, thus contributing to scientific research within the field of application. | | - DC
| The student is able to extract new knowledge and insights from datasets in the application domain. |
|
| EC = learning outcomes DC = partial outcomes BC = evaluation criteria |
|
The student should be familiar with statistical inference, statistical models, survival analysis.
The student should be familiar with programming in R.
|
|
|
Introduction & the basic SIR model (Kermack and McKendrick, 1927), Stochastic models & multiple subpopulations, Metapopulation models, Individual-based models, The analysis of serological data, The analysis of epidemic data, The analysis of surveillance data, Within-host models, Case studies I & II
|
|
|
Period 1 Credits 6,00
Evaluation method | |
|
|
Oral exam | 50 % |
|
Other | Questions about homeworks and project |
|
|
|
|
|
|
 
|
Compulsory course material |
|
The R software will be used in this course. |
|
 
|
Recommended reading |
|
- [Modeling Infectious Disease Parameters Based on Serological and Social Contact Data: A Modern Statistical Perspective],[Niel Hens; Ziv Shkedy; Marc Aerts; Christel Faes; Pierre Van Damme; Philippe Beutels],[],[Springer New York],[9781461440710],[Available as e-book: https://link.springer.com/book/10.1007%2F978-1-4614-4072-7]
- [Infectious Diseases of Humans: Dynamics and Control],[Roy M. Anderson; Robert M. May],[],[Oxford University Press],[9780198540403],[]
- [Handbook of Infectious Disease Data Analysis],[Leonhard Held, Niel Hens, Philip D O'Neill, Jacco Wallinga],[1st edition],[CRC Press],[9781032087351],[]
- [An Introduction to Infectious Disease Modelling],[Emilia Vynnycky, Richard White],[],[Oxford University Press, USA],[9780198565765],[]
|
|
 
|
Recommended course material |
|
R and Python will be used in this course.
|
|
|
|
|
|
1 Education, Examination and Legal Position Regulations art.12.2, section 2. |
2 Education, Examination and Legal Position Regulations art.16.9, section 2. |
3 Education, Examination and Legal Position Regulations art.15.1, section 3.
|
Legend |
SBU : course load | SP : ECTS | N : Dutch | E : English |
|