Language of instruction : English |
| Exam contract: not possible |
Sequentiality
|
|
No sequentiality
|
There is no data for this choice. Change the language, year or choose another item in the dropdown list if it is available.
| Degree programme | | Study hours | Credits | P2 SBU | P2 SP | 2nd Chance Exam1 | Tolerance2 | Final grade3 | |
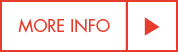 | 2nd year Master Quantitative Epidemiology | Compulsory | 81 | 3,0 | 81 | 3,0 | Yes | Yes | Numerical |  |
2nd year Master Quantitative Epidemiology - icp | Compulsory | 81 | 3,0 | 81 | 3,0 | Yes | Yes | Numerical |  |
|
| Learning outcomes |
- EC
| The student can handle scientific quantitative research questions, independently, effectively, creatively, and correctly using state-of-the-art design and analysis methodology and software. | | - DC
| ... correctly using state-of-the-art analysis methodology. | | - DC
| ... correctly using state-of-the-art software. | - EC
| The student is capable of acquiring new knowledge. | - EC
| The student routinely monitors his/her own learning process and adjusts and improves it accordingly. | - EC
| The student is able to efficiently acquire, store and process data. | | - DC
| ... selecting and using the best data management options | | - DC
| ...maintain provenance of data, analyses and results | - EC
| The student can critically appraise methodology and challenge proposals for and reported results of data analysis. | - EC
| The student has the habit to assess data quality and integrity. | - EC
| The student knows the international nature of the field of statistical science and data science. | - EC
| The student knows the societal relevance of statistics and data science. | | - DC
| The student can reflect on and explain the societal relevance of a task, particularly within the programme specialization | | - DC
| The student can reflect on societal tendencies, particularly within the programme specialization. | - EC
| The student knows the ethical, moral, legal, policy making, and privacy context of statistics and data science, and always acts accordingly. | | - DC
| The student can explain ethical issues and dilemmas within the fields of statistics and data science. | | - DC
| The student acts according to societal and ethical standards in general and particularly within the fields of statistics and data science. | | - DC
| The student respects the privacy of data, people and organizations with whom he/she comes into direct or indirect contact. | - EC
| The student is an effective written and oral communicator, both within their own field as well as across disciplines. | | - DC
| The student is an effective writer in their own field. | | - DC
| The student is an effective oral communicator in their own field. | - EC
| The student is able to correctly use the theory, either methodologically or in an application context or both, thus contributing to scientific research within the field of statistical science, data science, or within the field of application. | | - DC
| The student is able to correctly use the theory methodologically, thus contributing to scientific research within the field of statistical and data science.
| | - DC
| The student is able to correctly use the theory methodologically, thus contributing to scientific research within the field of application. | | - DC
| The student is able to correctly use the theory in an application context, thus contributing to scientific research within the field of statistical and data science.
| | - DC
| The student is able to correctly use the theory in an application context, thus contributing to scientific research within the field of application. | | - DC
| The student is able to extract new knowledge and insights from datasets in the application domain. |
|
| EC = learning outcomes DC = partial outcomes BC = evaluation criteria |
|
The pervasiveness of the Web and mobile technologies as well as the growing adoption of smart wearable sensors have significantly changed the landscape of epidemic intelligence data gathering with an unprecedented impact on global public health. The digital traces generated by a large number of individuals interacting with Web and mobile technologies as well as wearable sensors contain epidemiological indicators that are not easily accessed with traditional approaches. Collectively, these digital sources largely enhance the capabilities of epidemiology and global public health. Indeed, since more than a decade, the growing field of digital epidemiology (https://www.ncbi.nlm.nih.gov/pubmed/?term=29302758) has been using digital data generated outside the public health system to carry out epidemiological studies on an unprecedented scale and with an unprecedented precision. The goal of this course is to provide an overview of the main success stories collected during the first ten years of this young and exciting field. During the course, the students will be guided through popular methodologies and approaches in digital epidemiology with a focus on a few case studies with a hands-on approach.
|
|
|
|
|
|
|
Collective feedback moment ✔
|
|
|
Lecture ✔
|
|
|
Project ✔
|
|
|
Small group session ✔
|
|
|
|
Period 2 Credits 3,00
Evaluation method | |
|
Oral exam | 100 % |
|
|
Other | Questions about the content/materials of the final project. |
|
|
|
|
|
Second examination period
Evaluation second examination opportunity different from first examination opprt | |
|
|
 
|
Recommended course material |
|
A list of recommended reading papers will be communicated on Blackboard. |
|
|
|
|
|
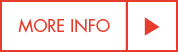 | 2nd year Master Biostatistics | Optional | 81 | 3,0 | 81 | 3,0 | Yes | Yes | Numerical |  |
2nd year Master Data Science | Optional | 81 | 3,0 | 81 | 3,0 | Yes | Yes | Numerical |  |
Exchange Programme Statistics | Optional | 81 | 3,0 | 81 | 3,0 | Yes | Yes | Numerical |  |
|
| Learning outcomes |
- EC
| The student can handle scientific quantitative research questions, independently, effectively, creatively, and correctly using state-of-the-art design and analysis methodology and software. | | - DC
| ... correctly using state-of-the-art analysis methodology. | | - DC
| ... correctly using state-of-the-art software. | - EC
| The student is capable of acquiring new knowledge. | - EC
| The student routinely monitors his/her own learning process and adjusts and improves it accordingly. | - EC
| The student is able to efficiently acquire, store and process data. | | - DC
| ... selecting and using the best data management options | | - DC
| ...maintain provenance of data, analyses and results | - EC
| The student can critically appraise methodology and challenge proposals for and reported results of data analysis. | - EC
| The student has the habit to assess data quality and integrity. | - EC
| The student knows the international nature of the field of statistical science and data science. | - EC
| The student knows the societal relevance of statistics and data science. | | - DC
| The student can reflect on and explain the societal relevance of a task, particularly within the programme specialization | | - DC
| The student can reflect on societal tendencies, particularly within the programme specialization. | - EC
| The student knows the ethical, moral, legal, policy making, and privacy context of statistics and data science, and always acts accordingly. | | - DC
| The student can explain ethical issues and dilemmas within the fields of statistics and data science. | | - DC
| The student acts according to societal and ethical standards in general and particularly within the fields of statistics and data science. | | - DC
| The student respects the privacy of data, people and organizations with whom he/she comes into direct or indirect contact. | - EC
| The student is an effective written and oral communicator, both within their own field as well as across disciplines. | | - DC
| The student is an effective writer in their own field. | | - DC
| The student is an effective oral communicator in their own field. | - EC
| The student is able to correctly use the theory, either methodologically or in an application context or both, thus contributing to scientific research within the field of statistical science, data science, or within the field of application. | | - DC
| The student is able to correctly use the theory methodologically, thus contributing to scientific research within the field of statistical and data science.
| | - DC
| The student is able to correctly use the theory methodologically, thus contributing to scientific research within the field of application. | | - DC
| The student is able to correctly use the theory in an application context, thus contributing to scientific research within the field of statistical and data science.
| | - DC
| The student is able to correctly use the theory in an application context, thus contributing to scientific research within the field of application. | | - DC
| The student is able to extract new knowledge and insights from datasets in the application domain. |
|
| EC = learning outcomes DC = partial outcomes BC = evaluation criteria |
|
The pervasiveness of the Web and mobile technologies as well as the growing adoption of smart wearable sensors have significantly changed the landscape of epidemic intelligence data gathering with an unprecedented impact on global public health. The digital traces generated by a large number of individuals interacting with Web and mobile technologies as well as wearable sensors contain epidemiological indicators that are not easily accessed with traditional approaches. Collectively, these digital sources largely enhance the capabilities of epidemiology and global public health. Indeed, since more than a decade, the growing field of digital epidemiology (https://www.ncbi.nlm.nih.gov/pubmed/?term=29302758) has been using digital data generated outside the public health system to carry out epidemiological studies on an unprecedented scale and with an unprecedented precision. The goal of this course is to provide an overview of the main success stories collected during the first ten years of this young and exciting field. During the course, the students will be guided through popular methodologies and approaches in digital epidemiology with a focus on a few case studies with a hands-on approach.
|
|
|
|
|
|
|
Collective feedback moment ✔
|
|
|
Lecture ✔
|
|
|
Project ✔
|
|
|
Small group session ✔
|
|
|
|
Period 2 Credits 3,00
Evaluation method | |
|
Oral exam | 100 % |
|
|
Other | Questions about the content/materials of the final project. |
|
|
|
|
|
|
 
|
Recommended course material |
|
A list of recommended reading papers will be communicated on Blackboard. |
|
|
|
|
|
1 Education, Examination and Legal Position Regulations art.12.2, section 2. |
2 Education, Examination and Legal Position Regulations art.16.9, section 2. |
3 Education, Examination and Legal Position Regulations art.15.1, section 3.
|
Legend |
SBU : course load | SP : ECTS | N : Dutch | E : English |
|