Language of instruction : English |
| Exam contract: not possible |
Sequentiality
|
|
No sequentiality
|
There is no data for this choice. Change the language, year or choose another item in the dropdown list if it is available.
| Degree programme | | Study hours | Credits | P2 SBU | P2 SP | 2nd Chance Exam1 | Tolerance2 | Final grade3 | |
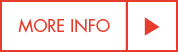 | 2nd year Master Data Science | Compulsory | 81 | 3,0 | 81 | 3,0 | Yes | Yes | Numerical |  |
|
| Learning outcomes |
- EC
| The student is capable of acquiring new knowledge. | - EC
| The student can critically appraise methodology and challenge proposals for and reported results of data analysis. |
|
| EC = learning outcomes DC = partial outcomes BC = evaluation criteria |
|
The student has basic knowledge in programming.
The student has basic knowledge of linear algebra such as eigenvalue problems and singular value decomposition
|
|
|
Five different topics by five different professors: Distributed Data Analysis, Frequent Itemset Handling, Gaussian Processes and Bayesian Optimization, Dimensionality Reduction, Numerical Linear Algebra.
Each of these topics will have a task exam and a lab work, which will have an equal weight. In order to pass the course, it is necessary that a minimum of 5/20 is obtained for each topic. A short oral assessment will be part of the evaluation.
|
|
|
|
|
|
|
Assignment ✔
|
|
|
Lecture ✔
|
|
|
Response lecture ✔
|
|
|
Self-study assignment ✔
|
|
|
|
|
|
Exercises ✔
|
|
|
Homework ✔
|
|
|
|
Period 2 Credits 3,00
Evaluation method | |
|
Written evaluaton during teaching periode | 75 % |
|
|
|
|
|
Second examination period
Evaluation second examination opportunity different from first examination opprt | |
|
|
 
|
Compulsory course material |
|
Different texts from the various modules. |
|
 
|
Recommended course material |
|
Course material will be provided via blackboard |
|
|
|
|
|
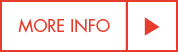 | Exchange Programme Statistics | Optional | 81 | 3,0 | 81 | 3,0 | Yes | Yes | Numerical |  |
|
|
|
The student has basic knowledge in programming.
The student has basic knowledge of linear algebra such as eigenvalue problems and singular value decomposition
|
|
|
Five different topics by five different professors: Distributed Data Analysis, Frequent Itemset Handling, Gaussian Processes and Bayesian Optimization, Dimensionality Reduction, Numerical Linear Algebra.
Each of these topics will have a task exam and a lab work, which will have an equal weight. In order to pass the course, it is necessary that a minimum of 5/20 is obtained for each topic. A short oral assessment will be part of the evaluation.
|
|
|
|
|
|
|
Assignment ✔
|
|
|
Lecture ✔
|
|
|
Response lecture ✔
|
|
|
Self-study assignment ✔
|
|
|
|
|
|
Exercises ✔
|
|
|
Homework ✔
|
|
|
|
Period 2 Credits 3,00
Evaluation method | |
|
Written evaluaton during teaching periode | 75 % |
|
|
|
|
|
Second examination period
Evaluation second examination opportunity different from first examination opprt | |
|
|
 
|
Compulsory course material |
|
Different texts from the various modules. |
|
 
|
Recommended course material |
|
Course material will be provided via blackboard |
|
|
|
|
|
1 Education, Examination and Legal Position Regulations art.12.2, section 2. |
2 Education, Examination and Legal Position Regulations art.16.9, section 2. |
3 Education, Examination and Legal Position Regulations art.15.1, section 3.
|
Legend |
SBU : course load | SP : ECTS | N : Dutch | E : English |
|