Language of instruction : English |
Exam contract: not possible |
Sequentiality
|
|
Mandatory sequentiality bound on the level of programme components
|
|
|
|
Following programme components must have been included in your study programme in a previous education period
|
|
|
Concepts of Probability and Statistics DL (3220)
|
5.0 stptn |
|
|
Linear Models DL (3577)
|
5.0 stptn |
|
|
| Degree programme | | Study hours | Credits | P1 SBU | P1 SP | 2nd Chance Exam1 | Tolerance2 | Final grade3 | |
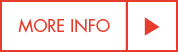 | second year Master Biostatistics - distance learning | Compulsory | 81 | 3,0 | 81 | 3,0 | Yes | Yes | Numerical |  |
second year Data Science - distance learning | Compulsory | 81 | 3,0 | 81 | 3,0 | Yes | Yes | Numerical |  |
|
| Learning outcomes |
- EC
| The student can handle scientific quantitative research questions, independently, effectively, creatively, and correctly using state-of-the-art design and analysis methodology and software. | | - DC
| ... correctly using state-of-the-art analysis methodology. | | - DC
| ... correctly using state-of-the-art design methodology. | - EC
| The student is capable of acquiring new knowledge. | - EC
| The student routinely monitors his/her own learning process and adjusts and improves it accordingly. | - EC
| The student can critically appraise methodology and challenge proposals for and reported results of data analysis. | - EC
| The student can work in a multidisciplinary, intercultural, and international team. | - EC
| The student knows the international nature of the field of statistical science and data science. | - EC
| The student knows the ethical, moral, legal, policy making, and privacy context of statistics and data science, and always acts accordingly. | | - DC
| The student can explain basic principles regarding ethics and integrity in general. | | - DC
| The student can explain ethical issues and dilemmas within the fields of statistics and data science. | - EC
| The student is an effective written and oral communicator, both within their own field as well as across disciplines. | | - DC
| The student is an effective writer in their own field. |
|
| EC = learning outcomes DC = partial outcomes BC = evaluation criteria |
|
The student knows the basics of statistical inference and probability and linear models.
|
|
|
In this course students will learn about some more advanced and state-of-the art statistical inference issues and techniques that are relevant for modern applications that go beyond the scope of traditional statistical methods:
- prediction versus association
- observational versus experimental studies
- basics of causal inference and causal machine learning.
Examples (with R code) will also be discussed.
|
|
|
|
|
|
|
Distance learning ✔
|
|
|
Project ✔
|
|
|
Q&A ✔
|
|
|
|
|
|
Group work ✔
|
|
|
Paper ✔
|
|
|
|
Period 1 Credits 3,00
Evaluation method | |
|
Written evaluaton during teaching periode | 50 % |
|
Transfer of partial marks within the academic year | ✔ |
|
|
|
|
|
|
|
Written exam | 30 % |
|
Transfer of partial marks within the academic year | ✔ |
|
|
|
|
|
|
|
|
Oral exam | 20 % |
|
Transfer of partial marks within the academic year | ✔ |
|
|
|
|
Other | Discussion about the paper |
|
|
|
|
|
Off campus online evaluation/exam | ✔ |
|
For the full evaluation/exam | ✔ |
|
|
|
Use of study material during evaluation | ✔ |
|
Explanation (English) | All course materials and own notations may be used. |
|
|
|
Evaluation conditions (participation and/or pass) | ✔ |
|
Conditions | To get a pass mark, the student must pass for each of the following parts: project, paper and oral exam. |
|
|
|
Consequences | If the condition is not met, the final mark will by the minimum of: - 9 - the total score of all evaluation components. |
|
|
|
|
 
|
Compulsory course material |
|
Course notes or slides will be made available on Blackboard. |
|
|
|
|
|
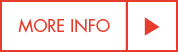 | second year Master Bioinformatics - distance learning | Optional | 81 | 3,0 | 81 | 3,0 | Yes | Yes | Numerical |  |
second year Quantitative Epidemiology - distance learning | Optional | 81 | 3,0 | 81 | 3,0 | Yes | Yes | Numerical |  |
|
| Learning outcomes |
- EC
| The student can handle scientific quantitative research questions, independently, effectively, creatively, and correctly using state-of-the-art design and analysis methodology and software. | | - DC
| ... correctly using state-of-the-art analysis methodology. | | - DC
| ... correctly using state-of-the-art design methodology. | - EC
| The student is capable of acquiring new knowledge. | - EC
| The student routinely monitors his/her own learning process and adjusts and improves it accordingly. | - EC
| The student can critically appraise methodology and challenge proposals for and reported results of data analysis. | - EC
| The student can work in a multidisciplinary, intercultural, and international team. | - EC
| The student knows the international nature of the field of statistical science and data science. | - EC
| The student knows the ethical, moral, legal, policy making, and privacy context of statistics and data science, and always acts accordingly. | | - DC
| The student can explain basic principles regarding ethics and integrity in general. | | - DC
| The student can explain ethical issues and dilemmas within the fields of statistics and data science. | - EC
| The student is an effective written and oral communicator, both within their own field as well as across disciplines. | | - DC
| The student is an effective writer in their own field. |
|
| EC = learning outcomes DC = partial outcomes BC = evaluation criteria |
|
The student knows the basics of statistical inference and probability and linear models.
|
|
|
In this course students will learn about some more advanced and state-of-the art statistical inference issues and techniques that are relevant for modern applications that go beyond the scope of traditional statistical methods:
- prediction versus association
- observational versus experimental studies
- basics of causal inference and causal machine learning.
Examples (with R code) will also be discussed.
|
|
|
|
|
|
|
Distance learning ✔
|
|
|
Project ✔
|
|
|
Q&A ✔
|
|
|
|
|
|
Group work ✔
|
|
|
Paper ✔
|
|
|
|
Period 1 Credits 3,00
Evaluation method | |
|
Written evaluaton during teaching periode | 50 % |
|
Transfer of partial marks within the academic year | ✔ |
|
|
|
|
|
|
|
Written exam | 30 % |
|
Transfer of partial marks within the academic year | ✔ |
|
|
|
|
|
|
|
|
Oral exam | 20 % |
|
Transfer of partial marks within the academic year | ✔ |
|
|
|
|
Other | Discussion about the paper |
|
|
|
|
|
Off campus online evaluation/exam | ✔ |
|
For the full evaluation/exam | ✔ |
|
|
|
Use of study material during evaluation | ✔ |
|
Explanation (English) | All course materials and own notations may be used. |
|
|
|
Evaluation conditions (participation and/or pass) | ✔ |
|
Conditions | To get a pass mark, the student must pass for each of the following parts: project, paper and oral exam. |
|
|
|
Consequences | If the condition is not met, the final mark will by the minimum of: - 9 - the total score of all evaluation components. |
|
|
|
Second examination period
Evaluation second examination opportunity different from first examination opprt | |
|
|
 
|
Compulsory course material |
|
Course notes or slides will be made available on Blackboard. |
|
|
|
|
|
1 Education, Examination and Legal Position Regulations art.12.2, section 2. |
2 Education, Examination and Legal Position Regulations art.16.9, section 2. |
3 Education, Examination and Legal Position Regulations art.15.1, section 3.
|
Legend |
SBU : course load | SP : ECTS | N : Dutch | E : English |
|