Language of instruction : English |
| Exam contract: not possible |
Sequentiality
|
|
Mandatory sequentiality bound on the level of programme components
|
|
|
Group 1 |
|
|
Following programme components must have been included in your study programme in a previous education period
|
|
|
Generalized Linear Models DL (3580)
|
3.0 stptn |
|
|
Linear Models DL (3577)
|
5.0 stptn |
|
|
Principles of Statistical Inference DL (3787)
|
3.0 stptn |
|
Or group 2 |
|
|
Following programme components must have been included in your study programme in a previous education period
|
|
|
Generalized Linear Models DL (3580)
|
6.0 stptn |
|
|
Linear Models DL (3577)
|
5.0 stptn |
|
|
Principles of Statistical Inference DL (3787)
|
3.0 stptn |
|
|
There is no data for this choice. Change the language, year or choose another item in the dropdown list if it is available.
| Degree programme | | Study hours | Credits | P1 SBU | P1 SP | 2nd Chance Exam1 | Tolerance2 | Final grade3 | |
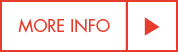 | second year Data Science - distance learning | Compulsory | 108 | 4,0 | 108 | 4,0 | Yes | Yes | Numerical |  |
|
| Learning outcomes |
- EC
| The student is capable of acquiring new knowledge. | - EC
| The student can critically appraise methodology and challenge proposals for and reported results of data analysis. | - EC
| The student can work in a multidisciplinary, intercultural, and international team. | - EC
| The student is an effective written and oral communicator, both within their own field as well as across disciplines. | - EC
| The student is able to correctly use the theory, either methodologically or in an application context or both, thus contributing to scientific research within the field of statistical science, data science, or within the field of application. |
|
| EC = learning outcomes DC = partial outcomes BC = evaluation criteria |
|
The student needs to have basic knowledge about principles of statistical inference, linear models, and generalized linear models.
|
|
|
The course is devoted to optimization and other numerical methods in the context of statistical modeling and other statistical computation. Chapter 2 introduces a number of motivating problems: the univariate model and the linear normal regression modes; this permits us to touch upon the least squares and maximum likelihood principles. Also, proportions, logistic regression, and contingency tables are introduced. Further topics involve: gamma regression, linear mixed models, generalized linear mixed models, principal components analysis, and cluster analysis. In Chapter 3, basic numerical tools are reviewed, such as Taylor series expansions and vector derivatives. The exponential family and a number of examples are considered. The basics of likelihood based inference are presented. In Chapter 4, we move from non-iterative to iterative procedures; this is done via estimation in the context of the normal and linear regression models on the one hand, and proportions and logistic regression on the other. Solving the score equations is considered, involving Newton-Raphson and Fisher-scoring. Finally, iterative reweighted least squares and the iterative proportional fitting algorithm are considered. The topic of Chapter 5 is least squares. The general principle is presented and applied to a variety of situations. These include closed forms and situations where iterative procedures are needed. Some extensions include: alternating/constrained/weighted least squares. Iteration-based function optimization is discussed in Chapter 6, including: the regula falsi, Newton’s method, and Newton-Raphson. The numerical calculation of derivatives is discussed, as well as the Nelder-Mead Simplex Algorithm. The MM algorithm is discussed in Chapter 7, together with a number of applications in regularized regression. Attention is given to lasso, elastic net, smooth regression, and regularized PCA. Chapter 8 is concerned with constrained optimization. A number of situations tackled are: variance estimation, success probabilities in binomial models, finite mixtures, and mixed models. Lagrange multipliers are discussed as well. An overview of maximum likelihood estimation and ensuing inferences is given in Chapter 9. This includes Wald and likelihood ratio tests. The delta method is described as well. Chapter 10 treats numerical integration, specifically in the context of mixed models and generalized linear mixed models. Precisely, attention is given to Gaussian quadrature and adaptive Gaussian quadrature. A wholesome treatment of the Expectation-Maximization algorithm can be found in Chapter 11. The EM algorithm is described through a number of applications on the one hand and via a more in-depth treatment on the other. Chapter 12 treats Monte Carlo methods for Bayesian computation. This includes Monte Carlo integration and Markov Chain Monte Carlo methods.
|
|
|
|
|
|
|
Collective feedback moment ✔
|
|
|
Distance learning ✔
|
|
|
Project ✔
|
|
|
|
Period 1 Credits 4,00
|
Off campus online evaluation/exam | ✔ |
|
For the full evaluation/exam | ✔ |
|
|
|
Additional information | An assignment is given prior to the exam, to be delivered in the form of a report just prior to the exam. It accounts for 50% of the total score. Students can work alone or in groups of two. At the oral exam, the students give an individual presentation (5 minutes) which accounts for 25% of the total score. It is followed by Q&A that accounts for 25% of the total score. |
|
Second examination period
Evaluation second examination opportunity different from first examination opprt | |
|
|
 
|
Compulsory course material |
|
The courses notes, as well as web lectures, are made available by the lecturers via BlackBoard. Hence, there is no need for a purchase via the bookshop |
|
|
|
|
|
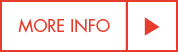 | second year Master Biostatistics - distance learning | Optional | 108 | 4,0 | 108 | 4,0 | Yes | Yes | Numerical |  |
second year Quantitative Epidemiology - distance learning | Optional | 108 | 4,0 | 108 | 4,0 | Yes | Yes | Numerical |  |
|
| Learning outcomes |
- EC
| The student is capable of acquiring new knowledge. | - EC
| The student can critically appraise methodology and challenge proposals for and reported results of data analysis. | - EC
| The student can work in a multidisciplinary, intercultural, and international team. | - EC
| The student is an effective written and oral communicator, both within their own field as well as across disciplines. | - EC
| The student is able to correctly use the theory, either methodologically or in an application context or both, thus contributing to scientific research within the field of statistical science, data science, or within the field of application. |
|
| EC = learning outcomes DC = partial outcomes BC = evaluation criteria |
|
The student needs to have basic knowledge about principles of statistical inference, linear models, and generalized linear models.
|
|
|
The course is devoted to optimization and other numerical methods in the context of statistical modeling and other statistical computation. Chapter 2 introduces a number of motivating problems: the univariate model and the linear normal regression modes; this permits us to touch upon the least squares and maximum likelihood principles. Also, proportions, logistic regression, and contingency tables are introduced. Further topics involve: gamma regression, linear mixed models, generalized linear mixed models, principal components analysis, and cluster analysis. In Chapter 3, basic numerical tools are reviewed, such as Taylor series expansions and vector derivatives. The exponential family and a number of examples are considered. The basics of likelihood based inference are presented. In Chapter 4, we move from non-iterative to iterative procedures; this is done via estimation in the context of the normal and linear regression models on the one hand, and proportions and logistic regression on the other. Solving the score equations is considered, involving Newton-Raphson and Fisher-scoring. Finally, iterative reweighted least squares and the iterative proportional fitting algorithm are considered. The topic of Chapter 5 is least squares. The general principle is presented and applied to a variety of situations. These include closed forms and situations where iterative procedures are needed. Some extensions include: alternating/constrained/weighted least squares. Iteration-based function optimization is discussed in Chapter 6, including: the regula falsi, Newton’s method, and Newton-Raphson. The numerical calculation of derivatives is discussed, as well as the Nelder-Mead Simplex Algorithm. The MM algorithm is discussed in Chapter 7, together with a number of applications in regularized regression. Attention is given to lasso, elastic net, smooth regression, and regularized PCA. Chapter 8 is concerned with constrained optimization. A number of situations tackled are: variance estimation, success probabilities in binomial models, finite mixtures, and mixed models. Lagrange multipliers are discussed as well. An overview of maximum likelihood estimation and ensuing inferences is given in Chapter 9. This includes Wald and likelihood ratio tests. The delta method is described as well. Chapter 10 treats numerical integration, specifically in the context of mixed models and generalized linear mixed models. Precisely, attention is given to Gaussian quadrature and adaptive Gaussian quadrature. A wholesome treatment of the Expectation-Maximization algorithm can be found in Chapter 11. The EM algorithm is described through a number of applications on the one hand and via a more in-depth treatment on the other. Chapter 12 treats Monte Carlo methods for Bayesian computation. This includes Monte Carlo integration and Markov Chain Monte Carlo methods.
|
|
|
|
|
|
|
Collective feedback moment ✔
|
|
|
Distance learning ✔
|
|
|
Project ✔
|
|
|
|
Period 1 Credits 4,00
|
Off campus online evaluation/exam | ✔ |
|
For the full evaluation/exam | ✔ |
|
|
|
Additional information | An assignment is given prior to the exam, to be delivered in the form of a report just prior to the exam. It accounts for 50% of the total score. Students can work alone or in groups of two. At the oral exam, the students give an individual presentation (5 minutes) which accounts for 25% of the total score. It is followed by Q&A that accounts for 25% of the total score. |
|
Second examination period
Evaluation second examination opportunity different from first examination opprt | |
|
|
 
|
Compulsory course material |
|
The courses notes, as well as web lectures, are made available by the lecturers via BlackBoard. Hence, there is no need for a purchase via the bookshop |
|
|
|
|
|
1 Education, Examination and Legal Position Regulations art.12.2, section 2. |
2 Education, Examination and Legal Position Regulations art.16.9, section 2. |
3 Education, Examination and Legal Position Regulations art.15.1, section 3.
|
Legend |
SBU : course load | SP : ECTS | N : Dutch | E : English |
|