Language of instruction : English |
Exam contract: not possible |
Sequentiality
|
|
No sequentiality
|
| Degree programme | | Study hours | Credits | P1 SBU | P1 SP | 2nd Chance Exam1 | Tolerance2 | Final grade3 | |
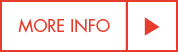 | second year Data Science - distance learning | Compulsory | 135 | 5,0 | 135 | 5,0 | Yes | Yes | Numerical |  |
|
| Learning outcomes |
- EC
| The student can handle scientific quantitative research questions, independently, effectively, creatively, and correctly using state-of-the-art design and analysis methodology and software. | | - DC
| ... correctly using state-of-the-art analysis methodology. | | - DC
| ... correctly using state-of-the-art software. | - EC
| The student is able to efficiently acquire, store and process data. | | - DC
| ... selecting and using the best data management options | | - DC
| ...maintain provenance of data, analyses and results | - EC
| The student is an effective written and oral communicator, both within their own field as well as across disciplines. | | - DC
| The student is an effective writer in their own field. | | - DC
| The student is an effective oral communicator in their own field. | - EC
| The student can put research and consulting aspects of one or more statistical fields into practice. | | - DC
| The student can put the research aspects of one or more statistical fields into practice.
| - EC
| The student is able to correctly use the theory, either methodologically or in an application context or both, thus contributing to scientific research within the field of statistical science, data science, or within the field of application. | | - DC
| The student is able to extract new knowledge and insights from datasets in the application domain. |
|
| EC = learning outcomes DC = partial outcomes BC = evaluation criteria |
|
This course aims to integrate knowledge and skills acquired in other courses (data management, programming courses, statistics and data visualisation). It takes the form of a group project assignment. No regular lectures are given, but rather a few seminars are organised. No new theory is provided by the seminars, but rather skills that are helpful for bringing the project assignment to a good end. With the project, students learn to integrate the different aspects of data science.
|
|
|
|
|
|
|
Collective feedback moment ✔
|
|
|
Project ✔
|
|
|
Response lecture ✔
|
|
|
|
Period 1 Credits 5,00
Evaluation method | |
|
Written evaluaton during teaching periode | 25 % |
|
|
|
|
|
Oral evaluation during teaching period | 25 % |
|
Transfer of partial marks within the academic year | ✔ |
|
|
|
|
|
|
|
|
Practical evaluation during teaching period | 50 % |
|
|
|
Off campus online evaluation/exam | ✔ |
|
For the full evaluation/exam | ✔ |
|
|
|
Evaluation conditions (participation and/or pass) | ✔ |
|
Conditions | The student must participate in all 3 parts of the evaluation : Github Code Bases, the practice evalution and the final project defense. The student must pass all three components in order to the course. |
|
|
|
Consequences | If the above conditions are not met, the final mark will by the minimum of: - 9 - the total score of all evaluation components. |
|
|
|
Second examination period
Evaluation second examination opportunity different from first examination opprt | |
|
Explanation (English) | If the student received a fail for the permanent practical evaluation a new individual project will be assigned. If the student receives a fail mark for the github repository or the project defense, the student needs to work on an individual basis to improve these components and needs to retake the project defense. |
|
|
|
|
 
|
Compulsory course material |
|
|
|
|
|
1 Education, Examination and Legal Position Regulations art.12.2, section 2. |
2 Education, Examination and Legal Position Regulations art.16.9, section 2. |
3 Education, Examination and Legal Position Regulations art.15.1, section 3.
|
Legend |
SBU : course load | SP : ECTS | N : Dutch | E : English |
|