Language of instruction : English |
Sequentiality
|
|
Advising sequentiality bound on the level of programme components
|
|
Advice
The student has preferably taken one of the following trajectory courses (2nd Ma Materiomics, Semester 1)
or: '4992 Molecular modelling - from principle to application in materials science' or: '4893 Density functional theory: the workhorse of first principles modelling of solids and molecules' or: '4894 Machine learning and artificial intelligence in modern materials science'
It is advised that this course is taken in tandem with the specialisation course '4904 New materials for photovoltaics'.
|
|
|
| Degree programme | | Study hours | Credits | P2 SBU | P2 SP | 2nd Chance Exam1 | Tolerance2 | Final grade3 | |
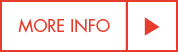 | 2nd year Master of Materiomics specialisatie opleidingsonderdelen | Optional | 81 | 3,0 | 81 | 3,0 | Yes | Yes | Numerical |  |
|
| Learning outcomes |
- EC
| EC 2. The graduate of the Master of Materiomics programme can combine chemical and physical principles enabling the discovery of new material concepts based on an interdisciplinary approach. | | - DC
| DC2.1 The student is able to design a structure with properties in mind. | | - DC
| DC2.2 The student is able to select and optimize a materials concept. | - EC
| EC 3. The graduate of the Master of Materiomics programme has insight in how modelling or synthesis methods predict and affect functional properties and is able to design sustainable materials based on in-operando functionality making optimal use of the synergy between computational and experimental methods. | | - DC
| DC3.4 The student is able to select, justify and optimize the appropriate characterization/modeling technique and method to investigate structure, synthesis, properties of materials and devices. | | - DC
| DC3.8 The student has knowledge of computational concepts and methods. [learning pathway interdisciplinarity - identification: the student knows which phenomena are studied in the various disciplines and which methods and theories are used] | - EC
| EC 4. The graduate of the Master of Materiomics programme is able to autonomously consult, summarise and critically interpret international scientific literature, reference it correctly and use it to explore and identify new domains relevant to the field. | | - DC
| DC4.2 The student is able to correctly and completely reference to scientific literature. | | - DC
| DC4.3 The student is able to critically interpret, evaluate, compare, and/or summarize relevant scientific literature related to materials-related problems or research questions. | - EC
| EC 6. The graduate of the Master of Materiomics programme is able to communicate in both written and spoken form and to take a well-argued position in a scientific discussion, going from a general to a specialist level, adapted to the target audience. | | - DC
| DC6.1 The student is able to report orally and in writing in an adequate manner. | | - DC
| DC6.3 The student is able to take and defend a logically constructed position, based on relevant and scientifically supported arguments. | - EC
| EC 10. The graduate of the Master of Materiomics programme is able to autonomously acquire new knowledge and monitor, evaluate and adjust one’s learning process. | | - DC
| DC10.3 The student is able to autonomously acquire, process, and critically interpret new information. | | - DC
| DC10.5 The student is able to relate new material-related interdisciplinary knowledge to his/her existing disciplinary knowledge base. [learning pathway interdisciplinarity - coordination: The student is able to make connections between different perspectives] |
|
| EC = learning outcomes DC = partial outcomes BC = evaluation criteria |
|
The student should have prior knowledge of the following general topics in physics/chemistry:
- basic concepts in quantum mechanics/chemistry
- basic concepts of chemical bonding and crystal structure
- basic knowledge of the electronic structure of molecules and solids
- basic concepts of statistics
- basic knowledge of scripting and an HPC environment (e.g. 4674 Fundamenten van materiaalmodellering (1Ma materiomics) )
This course extends on the student's knowledge obtained during the course "4674 Fundamenten van materiaalmodellering" (1Ma materiomics)
|
|
|
This specialisation course focuses on the predictive modelling of new materials with specific properties. The applications focus on energy materials in their broadest sense. However, it is important to note that the underlying methods are broadly applicable and therefore transferable to other applications as well. A central point in this specialisation course is modelling within the context of large and even gigantic amounts of information and datasets. Students come into contact with various methods for (a) dealing with these datasets (i.e. how to find a needle in a haystack) and (b) producing such datasets themselves (i.e. how to create the haystack yourself).
The topics covered during this course, within the context of application to energy materials, can be divided into the following two themes:
Data production
- What is "High-Throughput" and its role within big data
- High-performance computing and Lab robotics
- HT frameworks and thinking patterns
- Workflows, queues & databases
Data mining for material design & discovery
- Hierarchical screening of materials
- Material databases
- Principal Component Analysis & dimension reduction
- The role of Machine Learning within big data & high throughput based modelling
Within this course, students specialise their knowledge of computational modelling of materials with a view to designing new materials for energy (or other) applications. This design is rooted in the use of big data and high-throughput methodologies. Here, the student extends his/her own computational expertise. Learning goals of the course are:
- The student can select and/or identify relevant material parameters with the purpose of designing a new (energy) material
- The student has a clear picture of the role of big data and high-throughput within material design
- The student is familiar with existing materials databases and other sources of datasets
- The student develops a high-throughput based view on material design and understands the procedures followed in high-thoughput based research
- From a high-throughput thinking pattern, the student can translate a practical material design problem into efficient workflow that meets both physical/chemical and economic/ecological preconditions
- The student can place different computational methods within the workflow of big data & high-throughput based modelling, and knows the mutual interactions between the different methods
|
|
|
|
|
|
|
Lecture ✔
|
|
|
Response lecture/ journal club/ flipped classroom ✔
|
|
|
Video lectures with quiz ✔
|
|
|
|
|
|
Case study ✔
|
|
|
Presentation ✔
|
|
|
Seminar ✔
|
|
|
|
Period 2 Credits 3,00
Evaluation method | |
|
Evaluation method in consultation with student during teaching period | 30 % |
|
Transfer of partial marks within the academic year | ✔ |
|
Conditions transfer of partial marks within the academic year | The student achieves a minimum of 10/20. |
|
|
|
|
|
Explanation (English) | Continuous assessment includes an oral presentation and/or homework assignment and/or short paper assignments. The actual assignments are determined on the basis of the number of participating students and unique opportunities that arise during the teaching period. These assignment(s) are decided in consultation with the student(s). |
|
|
|
|
|
Written exam | 70 % |
|
Transfer of partial marks within the academic year | ✔ |
|
Conditions transfer of partial marks within the academic year | The student achieves a minimum of 10/20. |
|
|
|
|
|
|
|
|
|
|
Use of study material during evaluation | ✔ |
|
Explanation (English) | No internet-capable devices. |
|
|
|
Additional information | For students with an exam contract, permanent evaluation items during the teaching period are replaced by an alternative, individual assignment. |
|
Second examination period
Evaluation second examination opportunity different from first examination opprt | |
|
Explanation (English) | The written examination with oral explanation during the examination period may be retaken. Permanent evaluation items during the teaching period are replaced by an alternative, individual assignment. |
|
|
|
|
 
|
Compulsory course material |
|
Course notes, slides, selected (review) articles: available on Blackboard |
|
|
|
|
|
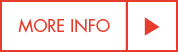 | Exchange Programme Chemistry | Optional | 81 | 3,0 | 81 | 3,0 | Yes | Yes | Numerical |  |
Exchange Programme materiomics | Optional | 81 | 3,0 | 81 | 3,0 | Yes | Yes | Numerical |  |
|
|
|
The student should have prior knowledge of the following general topics in physics/chemistry:
- basic concepts in quantum mechanics/chemistry
- basic concepts of chemical bonding and crystal structure
- basic knowledge of the electronic structure of molecules and solids
- basic concepts of statistics
- basic knowledge of scripting and an HPC environment (e.g. 4674 Fundamenten van materiaalmodellering (1Ma materiomics) )
This course extends on the student's knowledge obtained during the course "4674 Fundamenten van materiaalmodellering" (1Ma materiomics)
|
|
|
This specialisation course focuses on the predictive modelling of new materials with specific properties. The applications focus on energy materials in their broadest sense. However, it is important to note that the underlying methods are broadly applicable and therefore transferable to other applications as well. A central point in this specialisation course is modelling within the context of large and even gigantic amounts of information and datasets. Students come into contact with various methods for (a) dealing with these datasets (i.e. how to find a needle in a haystack) and (b) producing such datasets themselves (i.e. how to create the haystack yourself).
The topics covered during this course, within the context of application to energy materials, can be divided into the following two themes:
Data production
- What is "High-Throughput" and its role within big data
- High-performance computing and Lab robotics
- HT frameworks and thinking patterns
- Workflows, queues & databases
Data mining for material design & discovery
- Hierarchical screening of materials
- Material databases
- Principal Component Analysis & dimension reduction
- The role of Machine Learning within big data & high throughput based modelling
Within this course, students specialise their knowledge of computational modelling of materials with a view to designing new materials for energy (or other) applications. This design is rooted in the use of big data and high-throughput methodologies. Here, the student extends his/her own computational expertise. Learning goals of the course are:
- The student can select and/or identify relevant material parameters with the purpose of designing a new (energy) material
- The student has a clear picture of the role of big data and high-throughput within material design
- The student is familiar with existing materials databases and other sources of datasets
- The student develops a high-throughput based view on material design and understands the procedures followed in high-thoughput based research
- From a high-throughput thinking pattern, the student can translate a practical material design problem into efficient workflow that meets both physical/chemical and economic/ecological preconditions
- The student can place different computational methods within the workflow of big data & high-throughput based modelling, and knows the mutual interactions between the different methods
|
|
|
|
|
|
|
Lecture ✔
|
|
|
Response lecture/ journal club/ flipped classroom ✔
|
|
|
Video lectures with quiz ✔
|
|
|
|
|
|
Case study ✔
|
|
|
Presentation ✔
|
|
|
Seminar ✔
|
|
|
|
Period 2 Credits 3,00
Evaluation method | |
|
Evaluation method in consultation with student during teaching period | 30 % |
|
Transfer of partial marks within the academic year | ✔ |
|
Conditions transfer of partial marks within the academic year | The student achieves a minimum of 10/20. |
|
|
|
|
|
Explanation (English) | Continuous assessment includes an oral presentation and/or homework assignment and/or short paper assignments. The actual assignments are determined on the basis of the number of participating students and unique opportunities that arise during the teaching period. These assignment(s) are decided in consultation with the student(s). |
|
|
|
|
|
Written exam | 70 % |
|
Transfer of partial marks within the academic year | ✔ |
|
Conditions transfer of partial marks within the academic year | The student achieves a minimum of 10/20. |
|
|
|
|
|
|
|
|
|
|
Use of study material during evaluation | ✔ |
|
Explanation (English) | No internet-capable devices. |
|
|
|
Additional information | For students with an exam contract, permanent evaluation items during the teaching period are replaced by an alternative, individual assignment. |
|
Second examination period
Evaluation second examination opportunity different from first examination opprt | |
|
Explanation (English) | The written examination with oral explanation during the examination period may be retaken. Permanent evaluation items during the teaching period are replaced by an alternative, individual assignment. |
|
|
|
|
 
|
Compulsory course material |
|
Course notes, slides, selected (review) articles: available on Blackboard |
|
|
|
|
|
1 Education, Examination and Legal Position Regulations art.12.2, section 2. |
2 Education, Examination and Legal Position Regulations art.16.9, section 2. |
3 Education, Examination and Legal Position Regulations art.15.1, section 3.
|
Legend |
SBU : course load | SP : ECTS | N : Dutch | E : English |
|