Language of instruction : English |
Sequentiality
|
|
Advising sequentiality bound on the level of programme components
|
|
|
|
Following programme components are advised to also be included in your study programme up till now.
|
|
|
Process and Data Modelling (4851)
|
6.0 stptn |
|
|
There is no data for this choice. Change the language, year or choose another item in the dropdown list if it is available.
| Degree programme | | Study hours | Credits | P2 SBU | P2 SP | 2nd Chance Exam1 | Tolerance2 | Final grade3 | |
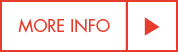 | Master of Management Data Science | Compulsory | 162 | 6,0 | 162 | 6,0 | Yes | Yes | Numerical |  |
|
| Learning outcomes |
- EC
| The holder of the degree is able to address complex management issues from a strategic and conceptual perspective by adapting and applying management paradigms in general, and in particular paradigms from their specialization. (Problem solving capacity) |
|
| EC = learning outcomes DC = partial outcomes BC = evaluation criteria |
|
In an era where data has become the new oil, understanding the role it plays in business decision-making is essential for every professional. This course is designed to equip learners with the fundamental knowledge of data science and its application to business decision-making.
The course begins with an overview of data-driven decision making, teaching learners to leverage data in the decision-making process. Students will be exposed to various types of data analytics – descriptive, predictive, and prescriptive – and understand how they contribute to interpreting past business performances, predicting future outcomes, and making optimal decisions. The course also introduces dashboards as a critical tool for presenting data insights and its role in a business intelligence context.
As we delve deeper, we will explore various methods and concepts of machine learning. By understanding these concepts, students will learn how machines can be trained to predict outcomes and identify patterns in a dataset, respectively. Subsequently, students wll also learn how to evaluate the quality of these analytical models. Here, learners will be introduced to metrics and techniques for assessing the performance and predictive power of these models.
By the end of this course, students will have a solid understanding of the role data science plays in business. They will be able to harness data to uncover insights, drive business strategies, and create impactful data narratives.
|
|
|
|
|
|
|
Lecture ✔
|
|
|
Response lecture ✔
|
|
|
Small group session ✔
|
|
|
|
Period 2 Credits 6,00
Evaluation method | |
|
Oral evaluation during teaching period | 30 % |
|
Transfer of partial marks within the academic year | ✔ |
|
|
|
|
|
|
|
Second examination period
Evaluation second examination opportunity different from first examination opprt | |
|
|
 
|
Compulsory textbooks (bookshop) |
|
[Data Science for Business: What you need to know about data mining and data-analytic thinking.],[Provost, Foster, and Tom Fawcett],[2013.],[O'Reilly Media, Inc.],[],[] |
|
 
|
Recommended course material |
|
Dit opleidingsonderdeel maakt gebruik van DataCamp For The Classroom, aangeboden door DataCamp (www.datacamp.com) |
|
|
|
|
|
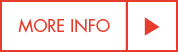 | Exchange Programme Business Economics | Optional | 162 | 6,0 | 162 | 6,0 | Yes | Yes | Numerical |  |
|
|
|
In an era where data has become the new oil, understanding the role it plays in business decision-making is essential for every professional. This course is designed to equip learners with the fundamental knowledge of data science and its application to business decision-making.
The course begins with an overview of data-driven decision making, teaching learners to leverage data in the decision-making process. Students will be exposed to various types of data analytics – descriptive, predictive, and prescriptive – and understand how they contribute to interpreting past business performances, predicting future outcomes, and making optimal decisions. The course also introduces dashboards as a critical tool for presenting data insights and its role in a business intelligence context.
As we delve deeper, we will explore various methods and concepts of machine learning. By understanding these concepts, students will learn how machines can be trained to predict outcomes and identify patterns in a dataset, respectively. Subsequently, students wll also learn how to evaluate the quality of these analytical models. Here, learners will be introduced to metrics and techniques for assessing the performance and predictive power of these models.
By the end of this course, students will have a solid understanding of the role data science plays in business. They will be able to harness data to uncover insights, drive business strategies, and create impactful data narratives.
|
|
|
|
|
|
|
Lecture ✔
|
|
|
Response lecture ✔
|
|
|
Small group session ✔
|
|
|
|
Period 2 Credits 6,00
Evaluation method | |
|
Oral evaluation during teaching period | 30 % |
|
Transfer of partial marks within the academic year | ✔ |
|
|
|
|
|
|
|
Second examination period
Evaluation second examination opportunity different from first examination opprt | |
|
|
 
|
Compulsory textbooks (bookshop) |
|
[Data Science for Business: What you need to know about data mining and data-analytic thinking.],[Provost, Foster, and Tom Fawcett],[2013.],[O'Reilly Media, Inc.],[],[] |
|
 
|
Recommended course material |
|
Dit opleidingsonderdeel maakt gebruik van DataCamp For The Classroom, aangeboden door DataCamp (www.datacamp.com) |
|
|
|
|
|
1 Education, Examination and Legal Position Regulations art.12.2, section 2. |
2 Education, Examination and Legal Position Regulations art.16.9, section 2. |
3 Education, Examination and Legal Position Regulations art.15.1, section 3.
|
Legend |
SBU : course load | SP : ECTS | N : Dutch | E : English |
|