Language of instruction : English |
Exam contract: not possible |
Sequentiality
|
|
Mandatory sequentiality bound on the level of programme components
|
|
|
Group 1 |
|
|
For following programme components you must have acquired a credit certificate, exemption, already tolerated unsatisfactory grade or selected tolerable unsatisfactory grade.
|
|
|
Concepts of Probability and Statistics DL (3220)
|
5,0 stptn |
|
|
Linear Models DL (3577)
|
5,0 stptn |
|
|
Programming in Python DL (3587)
|
5,0 stptn |
|
Or group 2 |
|
|
For following programme components you must have acquired a credit certificate, exemption, already tolerated unsatisfactory grade or selected tolerable unsatisfactory grade.
|
|
|
Concepts of Probability and Statistics DL (3220)
|
5,0 stptn |
|
|
Linear Models DL (3577)
|
5,0 stptn |
|
|
Programming in R DL (4432)
|
3,0 stptn |
|
|
| Degree programme | | Study hours | Credits | P1 SBU | P1 SP | 2nd Chance Exam1 | Tolerance2 | Final grade3 | |
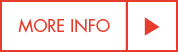 | second year Master Bioinformatics - distance learning | Compulsory | 135 | 5,0 | 135 | 5,0 | Yes | Yes | Numerical |  |
second year Data Science - distance learning | Compulsory | 135 | 5,0 | 135 | 5,0 | Yes | Yes | Numerical |  |
|
| Learning outcomes |
- EC
| The student can handle scientific quantitative research questions, independently, effectively, creatively, and correctly using state-of-the-art design and analysis methodology and software. | - EC
| The student is capable of acquiring new knowledge. | - EC
| The student is able to efficiently acquire, store and process data. | - EC
| The student can critically appraise methodology and challenge proposals for and reported results of data analysis. | - EC
| The student can work in a multidisciplinary, intercultural, and international team. | - EC
| The student knows the international nature of the field of statistical science and data science. | - EC
| The student is an effective written and oral communicator, both within their own field as well as across disciplines. |
|
| EC = learning outcomes DC = partial outcomes BC = evaluation criteria |
|
In this coursework we give a non-exhaustive overview of the basic principles of machine learning. In several classes we cover topics like, bias/variance trade off, simple linear regression and classification, cross validation and bootstrapping, unsupervised methods, feature selection methods, splines, random forests, and support vector machines. The theory is applied on a Kaggle competition. The course aims at junior level data scientists. Notion of programming and mathematics/statistics is mandatory.
- A framework for machine learning
- Simple supervised methods: Linear regression and Classification
- Resampling methods, Model selection and Regularization
- Moving beyond simple supervised methods:
- Regression splines
- Local regression
- Generalized additive models
- Tree-based method
- Support Vector Machines
- Unsupervised techniques
|
|
|
|
|
|
|
Flipped classroom ✔
|
|
|
Project ✔
|
|
|
Self-study assignment ✔
|
|
|
|
Period 1 Credits 5,00
Evaluation method | |
|
Written evaluaton during teaching periode | 25 % |
|
Transfer of partial marks within the academic year | ✔ |
|
Conditions transfer of partial marks within the academic year | The student needs to pass this component of evaluation. |
|
|
|
|
|
|
|
|
|
Oral evaluation during teaching period | 25 % |
|
Transfer of partial marks within the academic year | ✔ |
|
Conditions transfer of partial marks within the academic year | The student needs to pass this component of evaluation. |
|
|
|
|
|
|
|
|
|
|
Evaluation conditions (participation and/or pass) | ✔ |
|
Conditions | A student must at least attend all components of the evaluation.
A student must obtain a tolerable exam result (≥8/20) for each component to be able to pass the programme component. |
|
|
|
Consequences | If a student does not attend one of the evaluation components, he/she will receive an 'A - unauthorized absence' for the programme component.
If the student achieves less than 8/20 in part of this programme component, this lowest partial grade will be the final grade for the entire programme component for the examination opportunity concerned. |
|
|
|
Second examination period
Evaluation second examination opportunity different from first examination opprt | |
|
|
 
|
Prerequisites |
|
The student has knowledge about basic statistics and mathematics, such as, e.g., maximum likelihood estimation, linear regression, binary classification, matrix algebra, optimization. The student can program in R or Python. |
|
 
|
Compulsory textbooks (bookshop) |
|
An Introduction to Statistical Learning with Applications in R,James, G., Witten, D., Hastie, T. and Tibshirani, R.,2013,Springer-Verlag,(e-copy freely available online) |
|
 
|
Compulsory course material |
|
Lecture notes will be made available at Blackboard. |
|
 
|
Recommended reading |
|
The Elements of Statistical Learning,Hastie, T., Tibshirani, R. and Friedman, J.,2009,Springer-Verlag,Available as e-book: https://link.springer.com/book/10.1007%2F978-0-387-84858-7 |
|
|
|
|
|
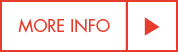 | second year Master Biostatistics - distance learning | Optional | 135 | 5,0 | 135 | 5,0 | Yes | Yes | Numerical |  |
second year Quantitative Epidemiology - distance learning | Optional | 135 | 5,0 | 135 | 5,0 | Yes | Yes | Numerical |  |
|
| Learning outcomes |
- EC
| The student can handle scientific quantitative research questions, independently, effectively, creatively, and correctly using state-of-the-art design and analysis methodology and software. | - EC
| The student is capable of acquiring new knowledge. | - EC
| The student is able to efficiently acquire, store and process data. | - EC
| The student can critically appraise methodology and challenge proposals for and reported results of data analysis. | - EC
| The student can work in a multidisciplinary, intercultural, and international team. | - EC
| The student knows the international nature of the field of statistical science and data science. | - EC
| The student is an effective written and oral communicator, both within their own field as well as across disciplines. |
|
| EC = learning outcomes DC = partial outcomes BC = evaluation criteria |
|
In this coursework we give a non-exhaustive overview of the basic principles of machine learning. In several classes we cover topics like, bias/variance trade off, simple linear regression and classification, cross validation and bootstrapping, unsupervised methods, feature selection methods, splines, random forests, and support vector machines. The theory is applied on a Kaggle competition. The course aims at junior level data scientists. Notion of programming and mathematics/statistics is mandatory.
- A framework for machine learning
- Simple supervised methods: Linear regression and Classification
- Resampling methods, Model selection and Regularization
- Moving beyond simple supervised methods:
- Regression splines
- Local regression
- Generalized additive models
- Tree-based method
- Support Vector Machines
- Unsupervised techniques
|
|
|
|
|
|
|
Flipped classroom ✔
|
|
|
Project ✔
|
|
|
Self-study assignment ✔
|
|
|
|
Period 1 Credits 5,00
Evaluation method | |
|
Written evaluaton during teaching periode | 25 % |
|
Transfer of partial marks within the academic year | ✔ |
|
Conditions transfer of partial marks within the academic year | The student needs to pass this component of evaluation. |
|
|
|
|
|
|
|
|
|
Oral evaluation during teaching period | 25 % |
|
Transfer of partial marks within the academic year | ✔ |
|
Conditions transfer of partial marks within the academic year | The student needs to pass this component of evaluation. |
|
|
|
|
|
|
|
|
|
|
Evaluation conditions (participation and/or pass) | ✔ |
|
Conditions | A student must at least attend all components of the evaluation.
A student must obtain a tolerable exam result (≥8/20) for each component to be able to pass the programme component. |
|
|
|
Consequences | If a student does not attend one of the evaluation components, he/she will receive an 'A - unauthorized absence' for the programme component.
If the student achieves less than 8/20 in part of this programme component, this lowest partial grade will be the final grade for the entire programme component for the examination opportunity concerned. |
|
|
|
|
 
|
Prerequisites |
|
The student has knowledge about basic statistics and mathematics, such as, e.g., maximum likelihood estimation, linear regression, binary classification, matrix algebra, optimization. The student can program in R or Python. |
|
 
|
Compulsory textbooks (bookshop) |
|
An Introduction to Statistical Learning with Applications in R,James, G., Witten, D., Hastie, T. and Tibshirani, R.,2013,Springer-Verlag,(e-copy freely available online) |
|
 
|
Compulsory course material |
|
Lecture notes will be made available at Blackboard. |
|
 
|
Recommended reading |
|
The Elements of Statistical Learning,Hastie, T., Tibshirani, R. and Friedman, J.,2009,Springer-Verlag,Available as e-book: https://link.springer.com/book/10.1007%2F978-0-387-84858-7 |
|
|
|
|
|
1 examination regulations art.1.3, section 4. |
2 examination regulations art.4.7, section 2. |
3 examination regulations art.2.2, section 3.
|
Legend |
SBU : course load | SP : ECTS | N : Dutch | E : English |
|