Language of instruction : English |
Exam contract: not possible |
Sequentiality
|
|
Mandatory sequentiality bound on the level of programme components
|
|
|
|
Following programme components must have been included in your study programme in a previous education period
|
|
|
Concepts of Probability and Statistics (1798)
|
5.0 stptn |
|
|
| Degree programme | | Study hours | Credits | P2 SBU | P2 SP | 2nd Chance Exam1 | Tolerance2 | Final grade3 | |
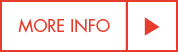 | 1st year Master Bioinformatics | Compulsory | 108 | 4,0 | 108 | 4,0 | Yes | No | Numerical |  |
1st year Master Bioinformatics - icp | Compulsory | 108 | 4,0 | 108 | 4,0 | Yes | No | Numerical |  |
1st year Master Biostatistics | Compulsory | 108 | 4,0 | 108 | 4,0 | Yes | No | Numerical |  |
1st year Master Biostatistics - icp | Compulsory | 108 | 4,0 | 108 | 4,0 | Yes | No | Numerical |  |
1st year Master Data Science | Compulsory | 108 | 4,0 | 108 | 4,0 | Yes | No | Numerical |  |
1st year Quantitative Epidemiology | Compulsory | 108 | 4,0 | 108 | 4,0 | Yes | No | Numerical |  |
1st year Master Quantitative Epidemiology - icp | Compulsory | 108 | 4,0 | 108 | 4,0 | Yes | No | Numerical |  |
|
| Learning outcomes |
- EC
| The student can handle scientific quantitative research questions, independently, effectively, creatively, and correctly using state-of-the-art design and analysis methodology and software. | | - DC
| ... correctly using state-of-the-art analysis methodology. | | - DC
| ... correctly using state-of-the-art software. | - EC
| The student is capable of acquiring new knowledge. | - EC
| The student can work in a multidisciplinary, intercultural, and international team. | - EC
| The student is an effective written and oral communicator, both within their own field as well as across disciplines. | | - DC
| The student is an effective writer in their own field. |
|
| EC = learning outcomes DC = partial outcomes BC = evaluation criteria |
|
This course will give a broad introduction to basic concepts of Bayesian analysis. Posterior summary measures, predictive distributions and Bayesian hypothesis tests will be contrasted with the frequentist approach. Simulation methods such as Markov chain Monte Carlo (MCMC) enable the Bayesian analysis. An introduction to algorithms like Gibbs sampling and Metropolis-Hastings will be explained and illustrated. Various medical case studies will be considered.
The student should be able to analyse relatively simple problems in a Bayesian way using OpenBugs, Nimble or JAGS software. The emphasis in this course is on theoretical background of basic concepts and practical data analysis.
|
|
|
|
|
|
|
Distance learning ✔
|
|
|
Lecture ✔
|
|
|
Project ✔
|
|
|
|
Period 2 Credits 4,00
Evaluation method | |
|
Written evaluaton during teaching periode | 30 % |
|
Transfer of partial marks within the academic year | ✔ |
|
Conditions transfer of partial marks within the academic year | score greater or equal to 10/20 on project |
|
|
|
|
|
Multiple-choice questions | ✔ |
|
|
|
|
|
|
Written exam | 70 % |
|
|
Multiple-choice questions | ✔ |
|
|
|
|
|
Second examination period
Evaluation second examination opportunity different from first examination opprt | |
|
Explanation (English) | Score for project is carried over to the retake exam (if score greater or equal to 10/20). Otherwise, a new project assignment has to be made. |
|
|
|
|
 
|
Compulsory textbooks (bookshop) |
|
Bayesian Biostatistics, Lesaffre, E. and Lawson, A., 2012, John Wiley & Sons,9780470018231 |
|
 
|
Compulsory course material |
|
All course material (handouts, video lectures and exercises) will be available on Blackboard. |
|
|
|
|
|
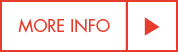 | Exchange Programme Biology | Optional | 108 | 4,0 | 108 | 4,0 | Yes | Yes | Numerical |  |
Exchange Programme Chemistry | Optional | 108 | 4,0 | 108 | 4,0 | Yes | Yes | Numerical |  |
Exchange Programme Physics | Optional | 108 | 4,0 | 108 | 4,0 | Yes | Yes | Numerical |  |
Exchange Programme Computer Science | Optional | 108 | 4,0 | 108 | 4,0 | Yes | Yes | Numerical |  |
Exchange Programme Statistics | Optional | 108 | 4,0 | 108 | 4,0 | Yes | Yes | Numerical |  |
Exchange Programme Mathematics | Optional | 108 | 4,0 | 108 | 4,0 | Yes | Yes | Numerical |  |
|
|
|
This course will give a broad introduction to basic concepts of Bayesian analysis. Posterior summary measures, predictive distributions and Bayesian hypothesis tests will be contrasted with the frequentist approach. Simulation methods such as Markov chain Monte Carlo (MCMC) enable the Bayesian analysis. An introduction to algorithms like Gibbs sampling and Metropolis-Hastings will be explained and illustrated. Various medical case studies will be considered.
The student should be able to analyse relatively simple problems in a Bayesian way using OpenBugs software. The emphasis in this course is on theoretical background of basic concepts and practical data analysis.
|
|
|
|
|
|
|
Distance learning ✔
|
|
|
Lecture ✔
|
|
|
Project ✔
|
|
|
|
Period 2 Credits 4,00
Evaluation method | |
|
Written evaluaton during teaching periode | 30 % |
|
Transfer of partial marks within the academic year | ✔ |
|
Conditions transfer of partial marks within the academic year | score greater or equal to 10/20 on project |
|
|
|
|
|
|
|
|
Written exam | 70 % |
|
|
Multiple-choice questions | ✔ |
|
|
|
|
|
Use of study material during evaluation | ✔ |
|
Explanation (English) | The students can make use of course slides, exercises and quizzes. |
|
|
|
Second examination period
Evaluation second examination opportunity different from first examination opprt | |
|
Explanation (English) | Score for project is carried over to the retake exam (if score greater or equal to 10/20). Otherwise, a new project assignment has to be made. |
|
|
|
|
 
|
Compulsory textbooks (bookshop) |
|
Bayesian Biostatistics, Lesaffre, E. and Lawson, A., 2012, John Wiley & Sons,9780470018231 |
|
 
|
Compulsory course material |
|
All course material (handouts, video lectures and exercises) will be available on Blackboard. |
|
|
|
|
|
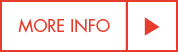 | 1st Master of Business and Information Systems Engineering | Optional | 108 | 4,0 | 108 | 4,0 | Yes | Yes | Numerical |  |
2nd Master of Business and Information Systems Engineering | Optional | 108 | 4,0 | 108 | 4,0 | Yes | Yes | Numerical |  |
|
| Learning outcomes |
- EC
| The holder of the degree shows autonomy in implementing scientific research methods. (Research skills) | - EC
| The holder of the degree shows autonomy in analysing, interpreting, evaluating and reporting research results. (Research skills) | - EC
| The holder of the degree uses data science and IT to design decision support systems that provide useful insights with which the quality of decisions can be improved. (Programme-specific competencies) |
|
| EC = learning outcomes DC = partial outcomes BC = evaluation criteria |
|
This course will give a broad introduction to basic concepts of Bayesian analysis. Posterior summary measures, predictive distributions and Bayesian hypothesis tests will be contrasted with the frequentist approach. Simulation methods such as Markov chain Monte Carlo (MCMC) enable the Bayesian analysis. An introduction to algorithms like Gibbs sampling and Metropolis-Hastings will be explained and illustrated. Various medical case studies will be considered.
The student should be able to analyse relatively simple problems in a Bayesian way using OpenBugs, Nimble or JAGS software. The emphasis in this course is on theoretical background of basic concepts and practical data analysis.
|
|
|
|
|
|
|
Distance learning ✔
|
|
|
Lecture ✔
|
|
|
Project ✔
|
|
|
|
Period 2 Credits 4,00
Evaluation method | |
|
Written evaluaton during teaching periode | 30 % |
|
Transfer of partial marks within the academic year | ✔ |
|
Conditions transfer of partial marks within the academic year | score greater or equal to 10/20 on project |
|
|
|
|
|
|
|
|
Written exam | 70 % |
|
|
Multiple-choice questions | ✔ |
|
|
|
|
|
Use of study material during evaluation | ✔ |
|
Explanation (English) | The students can make use of 4 pages of (own, written) summary during the exam. Other material is not allowed. |
|
|
|
Second examination period
Evaluation second examination opportunity different from first examination opprt | |
|
Explanation (English) | Score for project is carried over to the retake exam (if score greater or equal to 10/20). Otherwise, a new project assignment has to be made. |
|
|
|
|
 
|
Compulsory textbooks (bookshop) |
|
Bayesian Biostatistics, Lesaffre, E. and Lawson, A., 2012, John Wiley & Sons,9780470018231 |
|
 
|
Compulsory course material |
|
All course material (handouts, video lectures and exercises) will be available on Blackboard. |
|
|
|
|
|
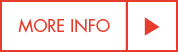 | Master of Teaching in Sciences and Technology - Engineering and Technology choice for subject didactics math | Optional | 108 | 4,0 | 108 | 4,0 | Yes | Yes | Numerical |  |
|
| Learning outcomes |
- EC
| WET 1. The newly graduated student has advanced knowledge, insight, skills and attitudes in the disciplines relevant to his/her specific subject didactics and is able to communicate these appropriately to his/her stakeholders. |
|
| EC = learning outcomes DC = partial outcomes BC = evaluation criteria |
|
This course will give a broad introduction to basic concepts of Bayesian analysis. Posterior summary measures, predictive distributions and Bayesian hypothesis tests will be contrasted with the frequentist approach. Simulation methods such as Markov chain Monte Carlo (MCMC) enable the Bayesian analysis. An introduction to algorithms like Gibbs sampling and Metropolis-Hastings will be explained and illustrated. Various medical case studies will be considered.
The student should be able to analyse relatively simple problems in a Bayesian way using OpenBugs, Nimble or JAGS software. The emphasis in this course is on theoretical background of basic concepts and practical data analysis.
|
|
|
|
|
|
|
Distance learning ✔
|
|
|
Lecture ✔
|
|
|
Project ✔
|
|
|
|
Period 2 Credits 4,00
Evaluation method | |
|
Written evaluaton during teaching periode | 30 % |
|
Transfer of partial marks within the academic year | ✔ |
|
Conditions transfer of partial marks within the academic year | score greater or equal to 10/20 on project |
|
|
|
|
|
|
|
|
Written exam | 70 % |
|
|
Multiple-choice questions | ✔ |
|
|
|
|
|
Use of study material during evaluation | ✔ |
|
Explanation (English) | The students can make use of course slides, exercises and quizzes. |
|
|
|
Second examination period
Evaluation second examination opportunity different from first examination opprt | |
|
Explanation (English) | Score for project is carried over to the retake exam (if score greater or equal to 10/20). Otherwise, a new project assignment has to be made. |
|
|
|
|
 
|
Compulsory textbooks (bookshop) |
|
Bayesian Biostatistics, Lesaffre, E. and Lawson, A., 2012, John Wiley & Sons,9780470018231 |
|
 
|
Compulsory course material |
|
All course material (handouts, video lectures and exercises) will be available on Blackboard. |
|
|
|
|
|
1 Education, Examination and Legal Position Regulations art.12.2, section 2. |
2 Education, Examination and Legal Position Regulations art.16.9, section 2. |
3 Education, Examination and Legal Position Regulations art.15.1, section 3.
|
Legend |
SBU : course load | SP : ECTS | N : Dutch | E : English |
|